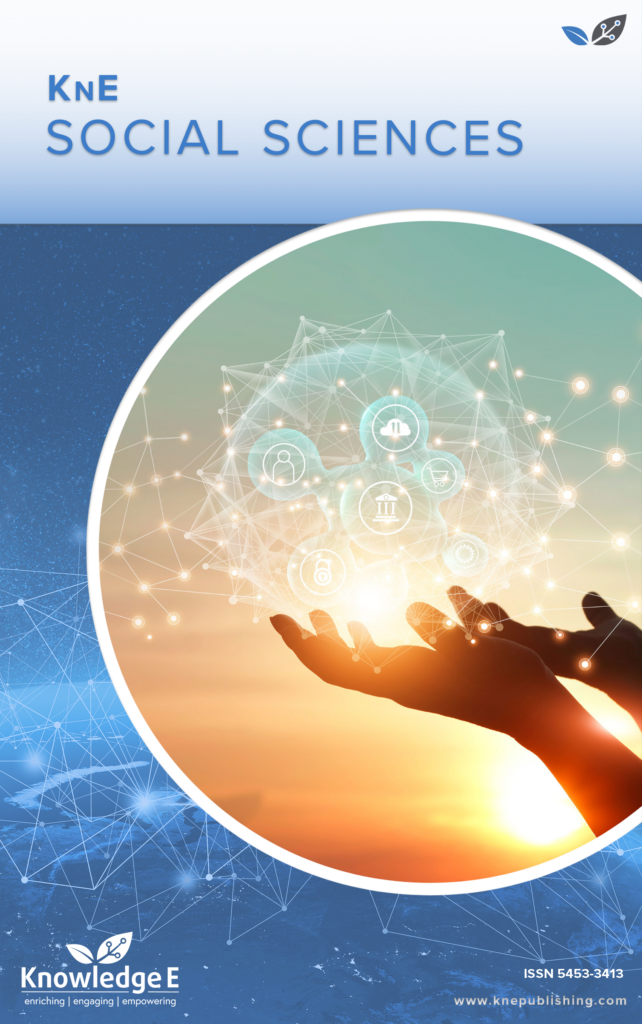
KnE Social Sciences
ISSN: 2518-668X
The latest conference proceedings on humanities, arts and social sciences.
Correlational Study of Mental Health and Achievement Among University Students
Published date:Aug 29 2024
Journal Title: KnE Social Sciences
Issue title: Annual Symposium on Applied Business Economics and Communication (ASABEC) 2023
Pages:154–165
Authors:
Abstract:
Mental health is a state of mental well-being that enables individuals to cope with life pressures, realize their capabilities, learn effectively, work proficiently, and contribute to their communities. According to UNICEF Indonesia, several conditions that affect a person’s mental health, including anxiety, depression, panic, and stress. Data shows that 1 out of 3 Indonesian teenagers experience mental health issues. These issues have spurred the present research to analyze the correlation between students’ mental health and their academic performance on campus. This study focuses on examining the correlation between mental health and the grade point average (GPA) of accounting students at the Jakarta State Polytechnic (PNJ). The research used a combination of eXtreme Gradient Boosting (XGBoost) and Shapley Additive Explanations (SHAP). This approach facilitates the analysis of the correlation between mental health and GPA among students at PNJ. In addition to its research sample, this methodology also constitutes an innovative aspect of the study. Based on the research conducted, the correlation between mental health indicators such as stress, anxiety, and depression and a decline in students’ GPA is found to be low. However, in extreme cases, feelings of anxiety and stress exhibit a negative correlation with GPA reduction in students.
Keywords: GPA, mental health, XGBoost, shap, correlation
References:
[1] World Health Organization. Mental health: Strengthening our response. World Health Organization; 2021.
[2] Center for Reproductive Health, University of Queensland, Johns Bloomberg Hopkins School of Public Health. Indonesia – National Adolescent Mental Health Survey (I-NAMHS): Laporan Penelitian. Pusat Kesehatan Reproduksi; 2022.
[3] Akhdan MA, Aminatun D. The correlation between anxiety and student gpa & Ept score during Covid 19 Pandemic. Journal of English Language Teaching and Learning. 2022;3(2):45–51.
[4] Hartley MT. Examining the relationships between resilience, mental health, and academic persistence in undergraduate college students. Journal of American college health. 2011;59(7):596–604.
[5] Hidayat DR. Bimbingan konseling: kesehatan mental di sekolah. Remaja Rosdakarya; 2013.
[6] Fusar-Poli P, de Pablo GS, De Micheli A, Nieman DH, Correll CU, Kessing LV, et al.What is good mental health? A scoping review. European neuropsychopharmacology. 2020;31:33–46.
[7] Massuhartono M, Mulyanti M. Terapi religi melalui dzikir pada penderita gangguan jiwa. Journal of Islamic Guidance and Counseling. 2018;2(2):201–14.
[8] Santrock JW. Educational psychology. McGraw-Hill; 2011.
[9] Nasrun MW, Kurniati A, Ulfiana E. Depresi, anxiety dan stres pada mahasiswa kedokteran dan hubungannya dengan indeks prestasi. Jurnal Pendidikan Kedokteran Indonesia. 2017;6(3):164–72.
[10] Fazakas-Dehoog L, Kuper A. Anxiety, depression, and academic performance: A study amongst Dutch and international students in the Netherlands. International Journal of Higher Education. 2018;7(2):72–83.
[11] Eisenberg D, Golberstein E, Hunt JB. Mental health and academic success in college. The BE journal of economic analysis & policy. 2009;9(1).
[12] Nunnally J, Bernstein I. Psychometric theory 3rd edition. New York: MacGraw-Hill; 1994.
[13] Yang Z, Chen J, Xue Y, Huang Z, Zheng J. Short-term electricity demand forecasting using gradient boosting decision tree based on improved feature selection. Energy and Buildings. 191AD;68–79.
[14] Zhang Y, Jia S, Zhou S, Xu H, Xu X. Forecasting the energy consumption of a district heating system using machine learning algorithms. Applied Energy. 2020;279:115901.
[15] Lundberg SM, Lee SI. A unified approach to interpreting model predictions. Advances in neural information processing systems. 2017;30.
[16] Lundberg SM, Erion GG, Lee SI. Consistent individualized feature attribution for tree ensembles. arXiv preprint arXiv:180203888. 2018.
[17] Adebayo J, Gilmer J, Muelly M, Goodfellow I, Hardt M, Kim B. Local explanations from causal models for machine learning. arXiv preprint arXiv:180510820. 2018.
[18] Jain S, Wallace BC. Attention is not explanation. arXiv preprint arXiv:190210186. 2019.