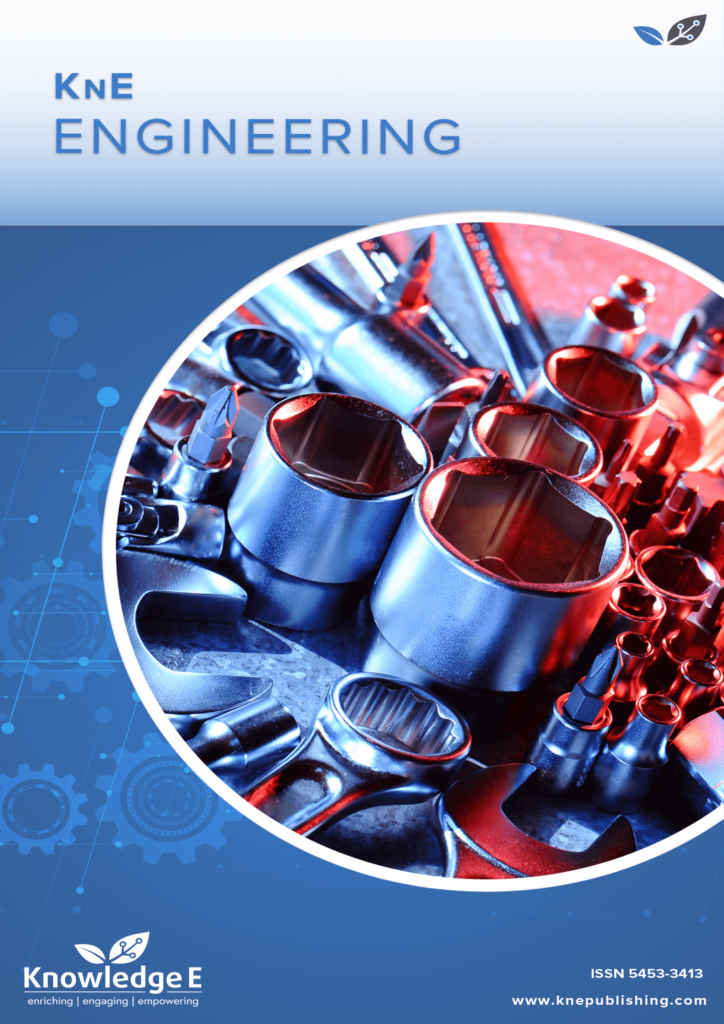
KnE Engineering
ISSN: 2518-6841
The latest conference proceedings on all fields of engineering.
The Application of Artificial Neural Networks in Predicting Structural Response of Multistory Building in The Region of Sumatra Island
Published date: Sep 05 2016
Journal Title: KnE Engineering
Issue title: Conference on Science and Engineering for Instrumentation, Environment and Renewable Energy
Pages:
Authors:
Abstract:
References:
[1] in FEMA 273 NEHRP guidelines for the seismic rehabilitation of buildings, 1, Federal Emergency Management Agency, Council, B.S.S., U.S.F.E.M. Agency, and A.T. Council, 1997.
[2] C. Yang, Study on Indonesian Seismic Code SNI 03-1726-2002 and Seismic Impact to High-rise Buildings in Jakarta, Indonesia, Proceedings of World Academy of Science: Engineering and Technology, p. 50, (2009).
[3] SNI-1726-2012, Standar Perencanaan Ketahanan Gempa Untuk Struktur Bangunan Gedung, 2012, Badan Standarisasi Nasional.
[4] D. M. Sahoo, A. Das, and S. Chakraverty, Interval data-based system identification of multistorey shear buildings by artificial neural network modelling, Archit Sci Rev, 1–11, (2014).
[5] M. Vafaei, A. Adnan, and A. B. Abd Rahman, Real-time Seismic Damage Detection of Concrete Shear Walls Using Artificial Neural Networks, J Earthquake Eng, 17, 137–154, (2012).
[6] T. Lay, H. Kanamori, C. J. Ammon, M. Nettles, S. N. Ward, R. C. Aster, S. L. Beck, S. L. Bilek, M. R. Brudzinski, R. Butler, H. R. DeShon, G. Ekström, K. Satake, and S. Sipkin, The great Sumatra-Andaman earthquake of 26 December 2004, Science, 308, 1127– 1133, (2005).
[7] S. Rajasekaran and G. AV. Pai, in Neural Network, Fuzzy logic, and Genetic Algorithms Syntesis and Applications, Prentice Hall of India, New Delhi, 2007.
[8] V. S. Kanwar, et al., Monitoring of RCC structures affected by earthquakes, Geomatics Nat Hazards Risk, 1–29, (2014).
[9] I. A. Basheer and M. Hajmeer, Artificial neural networks: fundamentals, computing, design, and application, J Microbiol Methods, 43, 3–31, (2000).