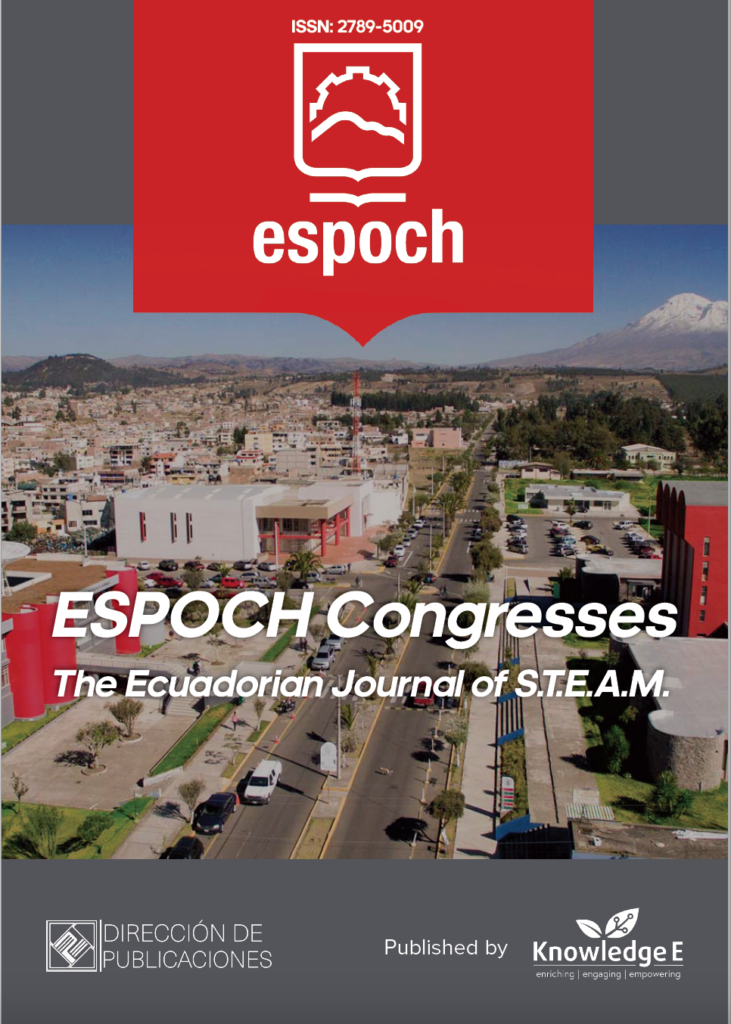
ESPOCH Congresses: The Ecuadorian Journal of S.T.E.A.M.
ISSN: 2789-5009
Leading Ecuadorian research in science, technology, engineering, arts, and mathematics.
Development of a Tool to Calculate the Preventive Maintenance Interval Using a Semi- Markovian Model Including a Degraded State
Published date:Jul 24 2024
Journal Title: ESPOCH Congresses: The Ecuadorian Journal of S.T.E.A.M.
Issue title: Volume 3, Issue 3
Pages:122 - 150
Authors:
Abstract:
This study aims to develop a tool that calculates the optimal preventive maintenance interval when the income from the operation of an asset changes. The income can be modified by market disturbances or by the decrease in the efficiency of the asset due to its degradation. A system with four states is designed to model the operation and maintenance process: operational, corrective, preventive, and degraded operation is mathematically modeled. The system evolves over time, according to a semi-Markovian process. The transitions and sojourn times between each state produce the accumulation of costs and income as returns (negative or positive) in a variable called average accumulated return. The average accumulated return is defined by a system of difference equations that are solved by applying the ztransform. The solution is a function that is dependent on the preventive interval. By derivation, the mathematical expression of the optimal preventive interval that maximizes the average accumulated return is obtained. From this expression, it can be deduced that the size of the optimal preventive interval is directly affected by the income from the asset operation. Higher income increases the size and lower income decreases it. For this reason, the maintenance manager must observe the changesoccuring in the income from the use of his equipment in order to optimize his management economically.
Keywords: preventive interval, income, semi-Markovian model, Wiener process.
Resumen
Se presenta una herramienta que calcula el intervalo de mantenimiento preventivo óptimo, cuando se modifica el ingreso obtenido por el funcionamiento de un activo. El ingreso se puede modificar por alteraciones del mercado, o por la disminución de la eficiencia del activo debido a su degradación. Para reflejar el proceso de operación y mantenimiento se modela matemáticamente un sistema con cuatro estados: operativo, correctivo, preventivo y operativo degradado. El sistema evoluciona en el tiempo, según un proceso semi-markoviano. Las transiciones y los tiempos de permanencia entre cada estado provocan la acumulación de costes e ingresos como retornos (negativos o positivos) en una variable llamada retorno medio acumulado. El retorno medio acumulado se define por un sistema de ecuaciones en diferencias que se resuelve aplicando la transformada z. La solución es una función que depende del intervalo preventivo y de la que por derivación se obtiene la expresión matemática del intervalo preventivo óptimo que maximiza el retorno medio acumulado. De esta expresión se deduce que el ingreso por el uso de un activo afecta directamente al tamaño del intervalo preventivo óptimo. Un mayor ingreso aumenta el tamaño y un menor ingreso lo disminuye. Por este motivo, el responsable de mantenimiento debe observar las modificaciones que se producen en los ingresos por el uso de sus equipos, con objeto de optimizar económicamente su gestión.
Palabras Clave: Intervalo preventivo, Ingresos, Modelo Semi-markoviano, Estado-degradado.
References:
[1] Yang L, Zhao Y, Peng R, Ma X. Hybrid preventive maintenance of competing failures under random environment. Reliab Eng Syst Saf. 2018;174:130–40.
[2] Zhong S, Pantelous AA, Goh M, Zhou J. A reliability-and-cost-based fuzzy approach to optimize preventive maintenance scheduling for offshore wind farms. Mech Syst Signal Process [Internet]. 2019;124:643-63. Disponible en: https://doi.org/10.1016/j.ymssp.2019.02.012.
[3] Lyubchenko A, Pacheco J, Kopytov E, Lutchenko S, Maystrenko V, Bartosh S. An approach for optimal maintenance planning of radio communication devices considering reliability and operational costs. En: Moscow Workshop on Electronic and Networking Technologies, MWENT 2018 - Proceedings. 2018. p. 1-5. https://doi.org/10.1109/MWENT.2018.8337301.
[4] de Jonge B, Scarf PA. A review on maintenance optimization. Eur J Oper Res. 2020;285(3):805–24.
[5] Młynarski S, Pilch R, Smolnik M, Szybka J, Wiązania G. A model of an adaptive strategy of preventive maintenance of complex technical objects. Eksploat i Niezawodn. 2020;22(1):35–41.
[6] Legát V, Mošna F, Aleš Z, Jurča V. Preventive maintenance models – higher operational reliability. Eksploat i Niezawodn - Maint Reliab. 2016;19(1):134-41.
[7] Sembiring N, Panjaitan N, Angelita S. Design of preventive maintenance system using the reliability engineering and maintenance value stream mapping methods in PT. XYZ. IOP Conf Ser Mater Sci Eng. 2018;309(1). https://doi.org/10.1088/1757- 899X/309/1/012128.
[8] Hu J, Shen J, Shen L. Periodic preventive maintenance planning for systems working under a Markovian operating condition. Comput Ind Eng [Internet]. 2020;142( January):106291. Disponible en: https://doi.org/10.1016/j.cie.2020.106291.
[9] Wang B, Wang X, Bie Z, Judge PD, Wang X, Green TC. Reliability model of MMC considering periodic preventive maintenance. IEEE Trans Power Deliv. 2017;32(3):1535–1544.
[10] Zhao X, Chen M, Nakagawa T. Periodic replacement policies with shortage and excess costs. Ann Oper Res [Internet]. 2020; Disponible en: https://doi.org/10.1007/s10479-020-03566-z.
[11] Zhao X, Cai J, Mizutani S, Nakagawa T. Preventive replacement policies with time of operations, mission durations, minimal repairs and maintenance triggering approaches. J Manuf Syst [Internet]. 2021;61:819-29. Disponible en: https://doi.org/10.1016/j.jmsy.2020.04.003.
[12] Semaan NM, Yehia N. A stochastic detailed scheduling model for periodic maintenance of military rotorcraft. Aircr Eng Aerosp Technol. 2019;91(9):1195–204.
[13] Liu Q, Dong M, Frank Chen F, Liu W, Ye C. Multi-objective imperfect maintenance optimization for production system with an intermediate buffer. J Manuf Syst [Internet]. 2020;56( July):452-62. Disponible en: https://doi.org/10.1016/j.jmsy.2020.07.002.
[14] Ruschel E, Santos EAP, Loures E de FR. Industrial maintenance decision-making: A systematic literature review. J Manuf Syst [Internet]. 2017;45:180-194. Disponible en: https://doi.org/10.1016/j.jmsy.2017.09.003.
[15] Kumar G, Varghese JP. Optimum preventive maintenance policy for a mechanical system using Semi-Markov method and Golden section technique. En: 2018 IEEE International Conference on Industrial Engineering and Engineering Management (IEEM). Bangkok; 2018. p. 232-236.
[16] Yi H, Cui L, Shen J, Li Y. Stochastic properties and reliability measures of discretetime semi-Markovian systems. Reliab Eng Syst Saf. 2017 May;2018(176):162–173.
[17] Wu B, Maya BI, Limnios N. Using Semi-Markov chains to solve semi-markov processes. Methodol Comput Appl Probab. 2021;23(4):1419–1431.
[18] Farahani A, Tohidi H, Shoja A. An integrated optimization of quality control chart parameters and preventive maintenance using Markov chain. Adv Prod Eng Manag. 2019;14(1):5–14.
[19] Gu X, Guo W, Jin X. Performance evaluation for manufacturing systems under control-limit maintenance policy. J Manuf Syst. 2020;55(March):221–32.
[20] Zhu X, Zhao F, Li J, Bai Y, Hu Q. Maintenance optimization based on three-stage failure process under performance-based contracting. Math Probl Eng. 2021;2021. https://doi.org/10.1155/2021/6323844.
[21] Mizutani S, Zhao XN. WIB (Which-Is-Better) Problems in maintenance reliability policies. In: Misra KB, editor. Handbook of Advanced Performability Engineering. Cham: Springer; 2021. pp. 523–47.
[22] Sánchez Herguedas A, Crespo Márquez A, Rodrigo Muñoz F. Optimizing preventive maintenance over a finite planning horizon in a semi-Markov framework. IMA J Manag Math [Internet]. 2022;33(1):75-99. Disponible en: https://doi.org/10.1093/imaman/dpaa026.
[23] Sánchez-Herguedas A, Mena-Nieto A, Rodrigo-Muñoz F. A new analytical method to optimise the preventive maintenance interval by using a Semi-Markov process and z-transform with an application to marine diesel engines. Reliab Eng Syst Saf. 2021 Mar;2021(207):1–15.
[24] Sánchez-Herguedas A, Mena-Nieto A, Rodrigo-Muñoz F, Villalba-Díez J, Ordieres-Meré J. Optimisation of maintenance policies based on rightcensored failure data using a semi-Markovian approach. Sensors [Internet]. 2022;22(4)(1432):1-18. Disponible en: https://www.mdpi.com/1424-8220/22/4/1432 https://doi.org/10.3390/s22041432.
[25] Sánchez-Herguedas A, Crespo-Márquez A, Rodrigo-Muñoz F. Optimising the preventive maintenance interval using a Semi-Markov process, z-transform, andfinite planning horizon. En: González-Prida V, Márquez CAP, Márquez AC, editores. Cases on Optimizing the Asset Management Process [Internet]. 2022.a ed. Hershey, PA: IGI Global; 2022 [citado 13 de octubre de 2021]. p. 137-61. Disponible en: http://services.igi-global.com/resolvedoi/resolve.aspx?doi=10.4018/978-1-7998- 7943-5.ch006 https://doi.org/10.4018/978-1-7998-7943-5.ch006.
[26] Sánchez-Herguedas A, Mena-Nieto A, Rodrigo-muñoz F. Villalba-Díez J. Aplicación de enfoques Semimarkovianos a la mejora de políticas de mantenimiento predeterminado en activos industriales. En: Tomás de J. Mateo Sanguino. José Manuel Lozano Domínguez, Manuel Joaquín Redondo González, Iñaki Josep Fernández de Viana González MÁRR, editor. Actas de las IV Jornadas ScienCity 2021 Fomento de la Cultura Científica, Tecnológica y de Innovación en Ciudades Inteligentes. Huelva; 2022. p. 23-6.
[27] Kong X, Yang J, Li L. Remaining useful life prediction for degrading systems with random shocks considering measurement uncertainty. J Manuf Syst [Internet]. 2021;61(December 2020):782-98. Disponible en: https://doi.org/10.1016/j.jmsy.2021.05.019.
[28] Baklouti A, Mifdal L, Dellagi S, Chelbi A, Al Madina B, Tillila Q, et al. An optimal preventive maintenance policy for a solar photovoltaic system [Internet]. Sustainability (Basel). 2020;12(10):13.
[29] Pandey M, Zuo MJ, Moghaddass R. Selective maintenance scheduling over a finite planning horizon. Proc Inst Mech Eng Part O J Risk Reliab [Internet]. 16 de agosto de 2015;230(2):162-77. Disponible en: https://doi.org/10.1177/1748006X15598914.
[30] Vadalá F, Bacigalupo A, Lepidi M, Gambarotta L. Free and forced wave propagation in beam lattice metamaterials with viscoelastic resonators. Int J Mech Sci. 2020 Sep;2021:193.
[31] Mikhin D. Analytic discrete transparent boundary conditions for high-order Padé parabolic equations. Wave Motion. 2008;45(7-8):881–94.
[32] Genschel U, Meeker WQ. A comparison of maximum likelihood and median-rank regression for Weibull estimation. Qual Eng. 2010;22(4):236–55.
[33] Olteanu D, Freeman L. The evaluation of Median-Rank Regression and Maximum Likelihood Estimation techniques for a two-parameter Weibull distribution. Qual Eng [Internet]. 2010;22(4):256-72. Disponible en: https://www.tandfonline.com/action/journalInformation?journalCode=lqen20 https://doi.org/10.1080/08982112.2010.505219.
[34] Assis EM, Figueirôa Filho CL, Lima GC, Salles GM, Pinto A. Salles GM de O, Pinto A. Comparison between maintenance policies based on q-Weibull and Weibull models. Int J Qual Reliab Manage. 2022;39(1):258–79.
[35] Crespo Márquez A. Dynamic modelling for supply chain management: Dealing with front-end, back-end and integration issues. Springer London; 2010.