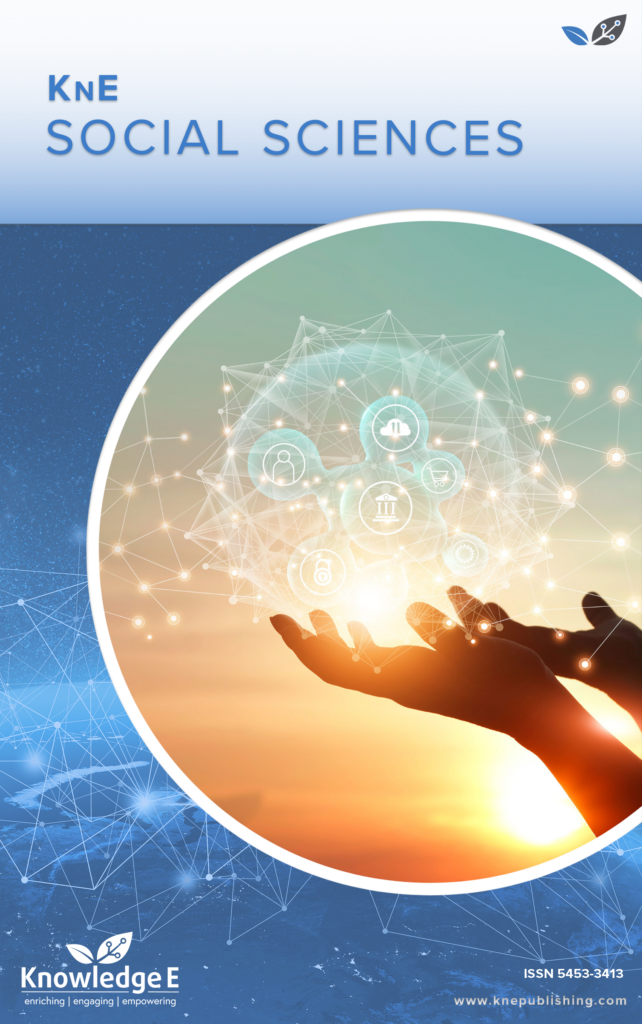
KnE Social Sciences
ISSN: 2518-668X
The latest conference proceedings on humanities, arts and social sciences.
Intelligent Data Management for Small Business Enhancement
Published date:Nov 19 2024
Journal Title: KnE Social Sciences
Issue title: The 1st International Conference on Creative Design, Business and Society (1st ICCDBS) 2023
Pages:9–24
Authors:
Abstract:
In the industry 4.0 era, there exists a pressing need for intelligent data management solutions to enhance the operations of small businesses. This study introduces a pioneering methodology that harnesses the power of AI-driven analysis of internal voice communications, an often-overlooked source of valuable insights within the small business environment. The research centers on an advanced platform that utilizes the Regularized Bayesian Approach, meticulously tailored for the processing of unstructured and semi-structured data, with a specific focus on internal voice messages. This methodology enables the generation of in-depth insights into employees’ emotional, psychological, and motivational states. Furthermore, the integration of data with a psychometric system enables the production of comprehensive personality evaluations, providing digital portraits for every employee. These portraits offer valuable insights into employee well-being and motivations, particularly beneficial for small businesses with limited HR resources. The potential benefits for small businesses are multifaceted and research-driven, including enhanced employee safety, improved efficiency, advanced risk management, and streamlined HR processes. Additionally, this research underscores the growing relevance and potential of this approach in the Emotion AI market. Through the analysis of voice messages, entities, intent, and relationships between utterances can be discerned, offering a comprehensive view of employee sentiment, loyalty, and satisfaction. This study serves as the foundation for fostering a positive work environment, enhancing productivity, and providing a roadmap for mental health improvement and reduced attrition in small businesses. It contributes to the evolving field of intelligent data management and its applications in enhancing small business operations.
Keywords: voice recognition, small business enhancement, emotion AI, artificial intelligence, Bayesian approach
References:
[1] Finkelstein L, Hofmann D. Intelligent measurement: a view of the state of art and current trends. Measurement. 1987;5(4):151–3.
[2] Hofmann D, Karaya K. Intelligent measurements for obtaining objective information in science and technology. In: X International Congress of IMECO; 1985; Prague. International Measurement Confederation (IMEKO).
[3] Rosenberg VY. Introduction to the theory of accuracy of measuring systems. Moscow: Sov. Radio; 1975.
[4] Michell J. Measurement in psychology: critical history of a methodological concept. Cambridge: Cambridge University Press; 1999. DOI: 10.1017/CBO9780511490040.
[5] Prokopchina SV. Development of methods and tools for Bayesian measurement intellectualization in complex object monitoring tasks. St. Petersburg; 1995.
[6] Prokopchina SV, Nedosekin DD, Chernyavsky EA. Information technologies of intellectualization of measuring processes. St. Petersburg: Energoatomizdat; 1995.
[7] Stevens SS. On the theory of the scales of measurement. Science. 1946;103(2684):677–80.
[8] Thurstone LL. A method of scaling psychological and educational tests. J Educ Psychol. 1925;16(7):16.
[9] von Hayek FA. The pretense of knowledge. Nobel Prize Lecture, December 11. Stockholm School of Economics.
[10] Guttman L. A basis for scaling qualitative data. Am Sociol Rev. 1944;9(2):139–50. DOI: 10.2307/2086306.
[11] Rasch G. Probabilistic model for some intelligence and attainment tests. Copenhagen: Danish Institute Educational Research; 1960.
[12] Andrich D. Rasch models for measurement. Thousand Oaks: Sage Publications; 1988.
[13] Mari L, Wilson M, Maul A. Measurement across the sciences: developing a shared concept system for measurement. New York: Springer; 2021.
[14] Giordani A, Mari L. A structural model of direct measurement. Measurement. 2019;145:535–50. DOI: 10.1016/j.measurement.2019.05.060.
[15] Finkelstein L. Widely, strongly and weakly defined measurement. Measurement. 2007;34(1):39–48.
[16] Wilson M. Constructing measures: an item response modeling approach. New York: Routledge; 2005.
[17] Holland P, Erlbaum. On sampling theory foundations of item response theory models. Psychometrika. 1990;55(4):577–601.
[18] International vocabulary of metrology – basic and general concepts and associated terms (VIM). Joint Committee for Guides in Metrology; 2012.
[19] Rossi GB. A probabilistic theory of measurement. Measurement. 2006;39:39–50.
[20] Rossi GB. Measurability. Measurement. 2007;40:545–62.
[21] Zaslavskaya VL, et al. Problems of using semistructured and unstructured data in business intelligence systems. S-Lib. 2022.