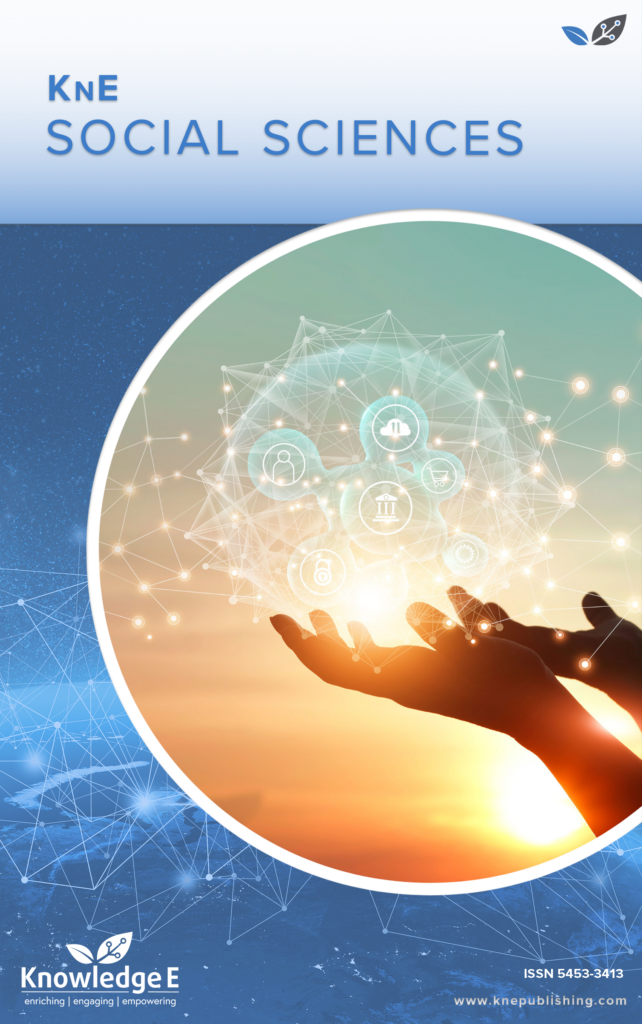
KnE Social Sciences
ISSN: 2518-668X
The latest conference proceedings on humanities, arts and social sciences.
Determining Appropriate Number of Labors in Yellow Noodle SMEs Using K-Means Clustering Method: Meeting Demand While Minimizing Company Costs
Published date: Oct 08 2024
Journal Title: KnE Social Sciences
Issue title: 4th International Conference in Social Science (4th ICONISS): Governance and Poverty Alleviation
Pages: 312–321
Authors:
Abstract:
Yellow noodle SMEs face the challenge of aligning their workforce with the fluctuating customer demand. Addressing this issue involves scaling up production staffing during peak yellow noodle demand. This study aimed to determine when and how much production labor should be added. Workforce expansion occurred through contractual arrangements during demand surges. This study used the K-Means clustering method to determine demand clusters. Each cluster underwent detailed analysis to calculate the precise number of required production workers. The study used the noodle sales in 2021–2022. Results showed that yellow noodle SMEs had eight permanent workers. Demand clusters were categorized into three classes. High-demand periods were observed in May and December, while moderate demand occurred in January, February, March, April, June, July, and August. September, October, and November constituted the low-demand cluster. Based on these findings, the study recommended adding one worker during high-demand and moderate-demand periods to maintain operational efficiency. Remarkably, no workforce additions were advised during the low-demand phase to optimize resource allocation and cost-effectiveness. In summary, this research addresses labor management challenges for yellow noodle SMEs. Leveraging data-driven approaches and cluster analysis offers SMEs a strategic framework to enhance workforce planning thereby improving operational efficiency and cost-effectiveness.
Keywords: yellow noodle SMEs, workforce demand clustering, operational efficiency
References:
[1] Kusuma TY, Firdaus MF. Penentuan Jumlah Tenaga Kerja Optimal Untuk Peningkatan Produktifitas Kerja (Studi Kasus : UD.Rekayasa Wangdi W). Integrated Lab Journal. 2019;7:26–36.
[2] Han J, Pei J, Tong H. Data Mining: Concepts and Techniques. 4th ed. Morgan Kaufmann; 2022.
[3] Harahap B. Penerapan Algoritma K-Means Untuk Menentukan Bahan Bangunan Laris (Studi Kasus Pada UD. Toko Bangunan YD Indarung). READY STAR - 2 2019;2:394– 403.
[4] Hutagalung J, Syahputra YH, Tanjung ZP. Pemetaan Siswa Kelas Unggulan Menggunakan Algoritma K-Means Clustering. Hal AH Nasution. 2022;9(1):606–20.
[5] Yudhistira A, Andika R. Pengelompokan Data Nilai Siswa Menggunakan Metode K-Means Clustering [ JAITI]. Journal of Artificial Intelligence and Technology Information. 2023;1(1):20–8.
[6] Abdullah D, Susilo S, Ahmar AS, Rusli R, Hidayat R. The application of K-means clustering for province clustering in Indonesia of the risk of the COVID-19 pandemic based on COVID-19 data. Qual Quant. 2022;56(3):1283–91.
[7] Khairani NA, Sutoyo E. Application of k-means clustering algorithm for determination of fire-prone areas utilizing hotspots in West Kalimantan Province. International Journal of Advances in Data and Information Systems. 2020;1(1):9–16.
[8] Harits D, Andriyas Puji A, Andivas M, Dermawan D, Thoriq EA, Raya JP, et al. Analisis Klaster Tingkat Pengangguran di Provinsi Kalimantan Timur Menggunakan Algoritma K-Means. Jurnal Surya Teknika. 2022;9(2):456–60.
[9] Sarbaini SW, Muttakin F. Cluster Analysis Menggunakan Algoritma Fuzzy K-Means Untuk Tingkat Pengangguran di Provinsi Riau [ JTMIT]. Jurnal Teknologi Dan Manajemen Industri Terapan. 2022;1(2):78–84.
[10] Shamrat FM, Tasnim Z, Mahmud I, Jahan N, Nobel NI. Application Of K-Means Clustering Algorithm To Determine The Density Of Demand Of Different Kinds Of Jobs. International Journal of Scientific & Technology Research. 2020;9:2.
[11] Astuti N, Utamajaya JN, Pratama A. Penerapan Data Mining Pada Penjualan Produk Digital Konter Leppangeng Cell Menggunakan Metode K-Means Clustering [ Jurnal Riset Komputer]. JURIKOM. 2022;9(3):754.
[12] Sallaby AF, Alinse RT, Sari VN, Ramadani T. Pengelompokan Barang Menggunakan Metode K-Means Clustering Berdasarkan Hasil PenjualanDi Toko Widya Bengkulu. Jurnal Media Infotama. 2022;18(1):99–104.
[13] Triyandana G, Putri LA, Umaidah Y. Penerapan Data Mining Pengelompokan Menu Makanan dan Minuman Berdasarkan Tingkat Penjualan Menggunakan Metode KMeans [ JAIC]. Journal of Applied Informatics and Computing. 2022;6(1):40–6.
[14] Hidayat T. Klasifikasi Data Jamaah Umroh Menggunakan Metode K-Means Clustering. Jurnal Sistim Informasi Dan Teknologi; 2022. pp. 19–24.
[15] Aldino AA, Darwis D, Prastowo AT, Sujana C. Implementation of K-Means Algorithm for Clustering Corn Planting Feasibility Area in South Lampung Regency. J Phys Conf Ser, vol. 1751, IOP Publishing Ltd; 2021.
[16] Kurniadewi H, Hakim RA, Jajuli M, Jaman JH. Pemetaan UMKM dalam Upaya Pengentasan Kemiskinan dan Penyerapan Tenaga Kerja Menggunakan Algoritma K-Means [ JAIC]. Journal of Applied Informatics and Computing. 2022;6(2):113–9.
[17] Rasyid R, Salim Y, Ramdaniah R. Sistem Informasi Pemetaan Kebutuhan Tenaga Kerja Guru berbasis Web menggunakan Metode K-Means. Buletin Sistem Informasi Dan Teknologi Islam. BUSITI; 2023. p. 4.
[18] Melani I, Priyatna B, Nurapriani F, Hilabi SS. Implementasi Metode K-Means Clustering Pada Penilaian Kinerja Karyawan PT Kopetri Citra Abadi. Jurnal Informasi Interaktif. 2023;8:24–30.
[19] Septiana V, Rahman AN. Penerapan Metode K-Means Clustering Untuk Mengelompokan Jenis Industri di Kota Tangerang Selatan. Math Vis. 2022;04(2):100–5.
[20] Mohammed J. Zaki, Wagner Meira Jr. Data Mining and Machine Learning: Fundamental Concepts and Algorithms. 2nd ed. Cambridge University Press; 2020.