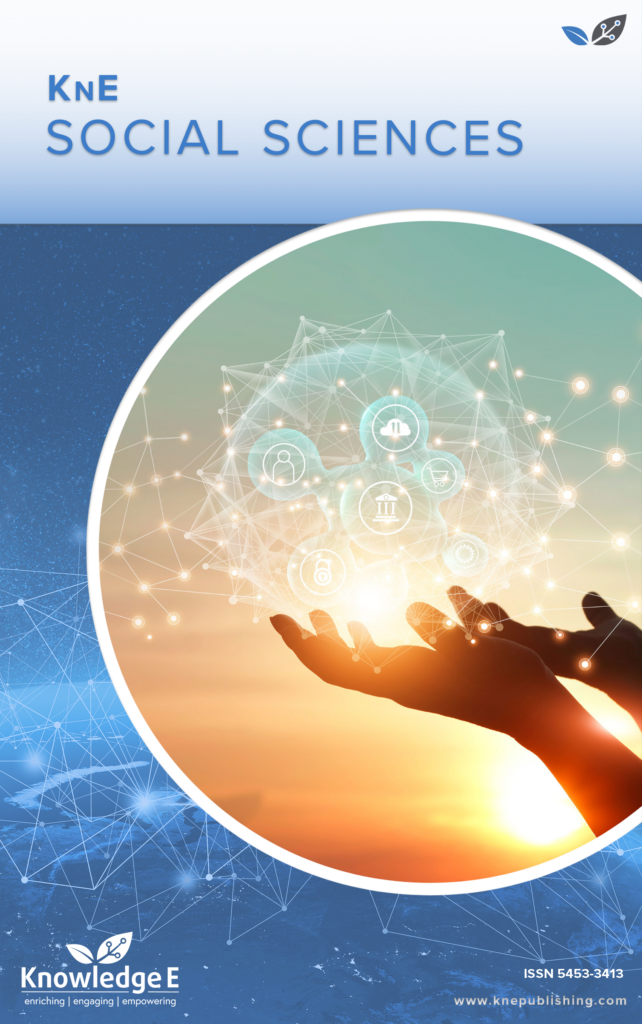
KnE Social Sciences
ISSN: 2518-668X
The latest conference proceedings on humanities, arts and social sciences.
Artificial Intelligence Driven Approaches for Financial Fraud Detection: A Systematic Literature Review
Published date: Jul 04 2024
Journal Title: KnE Social Sciences
Issue title: The 3rd Jakarta Economic Sustainability International Conference (3rd JESICA)
Pages: 448–468
Authors:
Abstract:
The primary aim of this research is to present a thorough and all-encompassing examination of artificial intelligence (AI) methodologies employed in the detection of financial fraud. The present study employs a systematic literature review (SLR) that was conducted utilizing the PRISMA approach. A comprehensive search was undertaken on reputable academic databases including ScienceDirect, Scopus, Springer, and Emerald, yielding a total of 24 papers published throughout the timeframe of 2014 to 2023. These articles will, thereafter, undergo further analysis. The findings of this study demonstrate that the implementation of artificial intelligence (AI) techniques for detecting financial fraud yields favorable outcomes. Specifically, the AI approach proves to be effective in enhancing the precision and efficiency of fraud pattern identification, thereby making a substantial contribution in this domain. In contrast, the prevailing methodology employed in the realm of financial fraud detection is frequently centered around machine learning. Furthermore, a majority of the research encompassed a diverse range of industries, with particular emphasis on the financial industry as the primary domain for the implementation of artificial intelligence (AI) in the detection of financial fraud.
Keywords: artificial intelligent, financial fraud, fraud detection
References:
[1] Sudarmanto E. Manajemen risiko: Deteksi dini upaya pencegahan fraud [Risk management: Early detection of fraud prevention efforts]. Jurnal Ilmu Manajemen. 2020;9(2):107-121. https://doi.org/10.32502/jimn.v9i2.2506. (Indonesian)
[2] Li J, Li N, Xia T, Guo J. Textual analysis and detection of financial fraud: Evidence from Chinese manufacturing firms. Economic Modelling. 2023;126:106428. https://doi.org/10.1016/j.econmod.2023.106428
[3] Sun H, Li J, Zhu X. Financial fraud detection based on the part-of-speech features of textual risk disclosures in financial reports. Procedia Computer Science. 2023;221:57- 64. https://doi.org/10.1016/j.procs.2023.07.009
[4] Yan M, Filieri R, Gorton M. Continuance intention of online technologies: A systematic literature review. International Journal of Information Management. 2021;58:102315. https://doi.org/10.1016/j.ijinfomgt.2021.102315
[5] Pinto SO, Sobreiro VA. Literature review: Anomaly detection approaches on digital business financial systems. Digital Business. 2022;2(2):100038. https://doi.org/10.1016/j.digbus.2022.100038
[6] Zhang D, Frei R, Senyo PK, et al. Understanding fraudulent returns and mitigation strategies in multichannel retailing. Journal of Retailing and Consumer Services. 2023;70:103145. https://doi.org/10.1016/j.jretconser.2022.103145
[7] Abedini A, Salimi M, Mazaheri Y, et al. Assessment of cheese frauds, and relevant detection methods: A systematic review. Food Chemistry: X. 2023;19:100825. https://doi.org/10.1016/j.fochx.2023.100825
[8] Wyrobek J. Application of machine learning models and artificial intelligence to analyze annual financial statements to identify companies with unfair corporate culture. Procedia Computer Science. 2020;176:3037-3046. https://doi.org/10.1016/j.procs.2020.09.335
[9] Achituve I, Kraus S, Goldberger J. Interpretable online banking fraud detection based on hierarchical attention mechanism. IEEE 29th International Workshop on Machine Learning for Signal Processing (MLSP) [Internet]; 2019 Oct 13-16; Pittsburgh, PA, USA. New Jersey: IEEE; 2019 [cited 2023 Jun 3]. 6 p. Available from: https://doi.org/10.1109/MLSP.2019.8918896
[10] Akmaluddin M, Dewayanto T. Systematic literature review: Implementasi artificial intelligence dan machine learning pada bidang akuntansi manajemen [Systematic literature review: Implementation of artificial intelligence and machine learning in the field of management accounting]. Diponegoro Journal of Accounting. 2023;12(4):1-11. (Indonesian)
[11] Cherif A, Badhib A, Ammar H, Alshehri S, Kalkatawi M, Imine A. Credit card fraud sdetection in the era of disruptive technologies: A systematic review. Journal of King Saud University-Computer and Information Sciences. 2023;35(1):145-174. https://doi.org/10.1016/j.jksuci.2022.11.008
[12] Murima WH, Prayogi ARY, Rahvy AP, Djunaedi N, Dhamanti I. Telemedicine use in health facility during covid-19 pandemic: Literature review. Indonesian Journal of Health Administration. 2022;10(2):251-260. https://doi.org/10.20473/jaki.v10i2.2022.251-260
[13] Lame G. Systematic literature reviews: An introduction. Proceedings of the Design Society: International Conference on Engineering Design. 2019;1(1):1633-1642. https://doi.org/10.1017/dsi.2019.169
[14] Page MJ, McKenzie JE, Bossuyt PM, et al. The PRISMA 2020 statement: An updated guideline for reporting systematic reviews. BMJ. 2021;372:n71. https://doi.org/10.1136/bmj.n71
[15] Osegi EN, Jumbo EF. Comparative analysis of credit card fraud detection in simulated annealing trained artificial neural network and hierarchical temporal memory. Machine Learning with Applications. 2021;6:100080. https://doi.org/10.1016/j.mlwa.2021.100080
[16] Domashova J, Zabelina O. Detection of fraudulent transactions using SAS Viya machine learning algorithms. Procedia Computer Science. 2021;190:204-209. https://doi.org/10.1016/j.procs.2021.06.025
[17] Lucas Y, Portier P-E, Laporte L, et al. Towards automated feature engineering for credit card fraud detection using multi-perspective HMMs. Future Generation Computer Systems. 2020;102:393-402. https://doi.org/10.1016/j.future.2019.08.029
[18] Lu Q, Fu C, Nan K, et al. Chinese corporate fraud risk assessment with machine learning. Intelligent Systems with Applications. 2023;20:200294. https://doi.org/10.1016/j.iswa.2023.200294
[19] Canhoto AI. Leveraging machine learning in the global fight against money laundering and terrorism financing: An affordances perspective. Journal of Business Research. 2021;131:441-452. https://doi.org/10.1016/j.jbusres.2020.10.012
[20] Zhu X, Qin XAZ, Chang Y, Liu Y, He Q, Li J. Intelligent financial fraud detection practices post- pandemic era. The Innovation. 2021;2(4):100176. https://doi.org/10.1016/j.xinn.2021.100176
[21] Moreira MÂL, Junior CSR, Silva DFL, et al. Exploratory analysis and implementation of machine learning techniques for predictive assessment of fraud in banking systems. Procedia Computer Science. 2022;214:117-124. https://doi.org/10.1016/j.procs.2022.11.156
[22] Lokanan ME, Sharma K. Fraud prediction using machine learning: The case of investment advisors in Canada. Machine Learning with Applications. 2022;8,:100269. https://doi.org/10.1016/j.mlwa.2022.100269
[23] Karunachandra B, Putera N, Wijaya SR, Suryani D, Wesley J, Purnama Y. On the benefits of machine learning classification in cashback fraud detection. Procedia Computer Science. 2023;216:364-369. https://doi.org/10.1016/j.procs.2022.12.147
[24] Chen L, Jia N, Zhao H, Kang Y, Deng J, Ma S. Refined analysis and a hierarchical multitask learning approach for loan fraud detection. Journal of Management Science and Engineering. 2022;7(4):589-607. https://doi.org/10.1016/j.jmse.2022.06.001
[25] Chen K, Yadav A, Khan A, Zhu K. Credit fraud detection based on hybrid credit scoring model. Procedia Computer Science. 2020;167:2-8. https://doi.org/10.1016/j.procs.2020.03.176
[26] Mao X, Liu M, Wang Y. Using GNN to detect financial fraud based on the related party transactions network. Procedia Computer Science. 2022;214:351-358. https://doi.org/10.1016/j.procs.2022.11.185
[27] Misra S, Thakur S, Gosh M, Saha SK. An autoencoder based model for detecting fraudulent credit card transaction. Procedia Computer Science. 2020;167:254-262. https://doi.org/10.1016/j.procs.2020.03.219
[28] Izotova A, Valiullin A. Comparison of Poisson process and machine learning algorithms approach for credit card fraud detection. Procedia Computer Science. 2021;186:721-726. https://doi.org/10.1016/j.procs.2021.04.214
[29] Arslan E, Güneş A. Fraud detection in enterprise resource planning systems using one-class support vector machine combined with convolutional neural network: The case of Spor Istanbul. Annals of Applied Sport Sciences. 2023;11(S1). http://dx.doi.org/10.61186/aassjournal.1222
[30] Chen S. Detection of fraudulent financial statements using the hybrid data mining approach. SpringerPlus. 2016;5:89. https://doi.org/10.1186/s40064-016-1707-6
[31] Ileberi E, Sun Y, Wang Z. A machine learning based credit card fraud detection using the GA algorithm for feature selection. Journal of Big Data. 2022;9:24. https://doi.org/10.1186/s40537-022-00573-8
[32] Li J. E-commerce fraud detection model by computer artificial intelligence data mining. Computational Intelligence and Neuroscience. 2022;2022:8783783. https://doi.org/10.1155/2022/8783783
[33] Lokanan M, Tran V, Vuong NH. Detecting anomalies in financial statements using machine learning algorithm: The case of Vietnamese listed firms. Asian Journal of Accounting Research, 2019;4(2):181-201. https://doi.org/10.1108/AJAR-09-2018-0032
[34] Patil S, Nemade V, Soni PK. Predictive modelling for credit card fraud detection using data analytics. Procedia Computer Science. 2018;132:385-395. https://doi.org/10.1016/j.procs.2018.05.199
[35] Halvaiee NS, Akbari MK. A novel model for credit card fraud detection using artificial immune systems. Applied Soft Computing. 2014;24:40-49. https://doi.org/10.1016/j.asoc.2014.06.042
[36] Lin C-C, Chiu A-A, Huang SY, Yen DC. Detecting the financial statement fraud: The analysis of the differences between data mining techniques and experts’ judgments. Knowledge-Based Systems. 2015;89:459-470. https://doi.org/10.1016/j.knosys.2015.08.011
[37] Chen Y-J, Wu C-H, Chen Y-M, Li H-Y, Chen H-K. Enhancement of fraud detection for narratives in annual reports. International Journal of Accounting Information Systems. 2017;26:32-45. https://doi.org/10.1016/j.accinf.2017.06.004