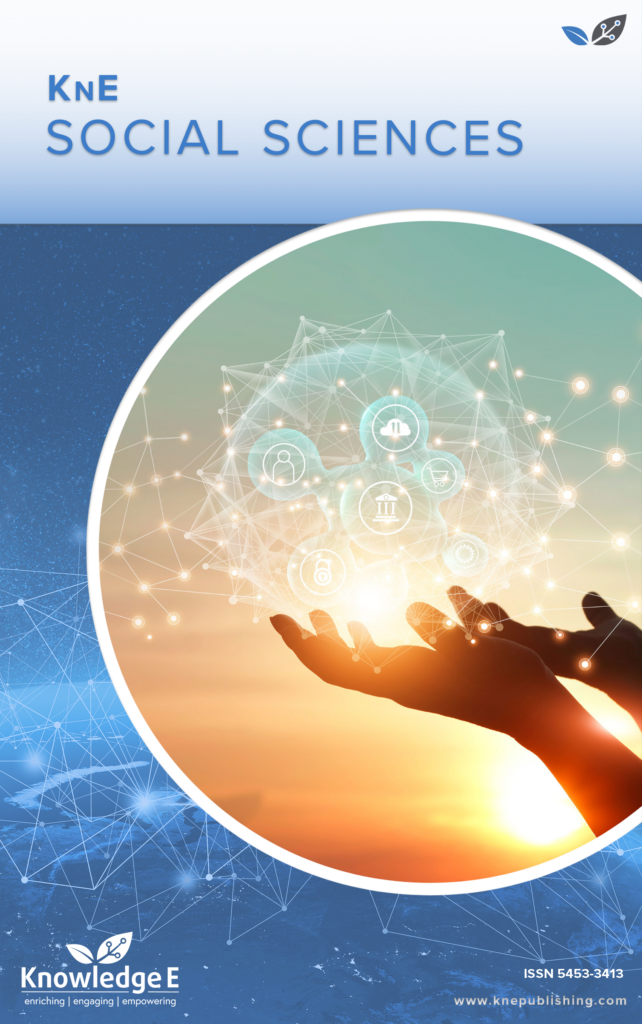
KnE Social Sciences
ISSN: 2518-668X
The latest conference proceedings on humanities, arts and social sciences.
Landslide Susceptibility Assessment in Mojokerto Regency Using Logistic Regression
Published date: Oct 12 2022
Journal Title: KnE Social Sciences
Issue title: 3rd International Conference on Geography and Education (ICGE)
Pages: 231–246
Authors:
Abstract:
The impact of landslides varies from place to place, including cutting off transportation routes, destroying agricultural land, and/or destroying houses. Due to the high threat of landslides, it is necessary to make efforts to improve community preparedness by disseminating information about landslide distribution. In this research, landslide assessment was conducted using logistic regression. Twelve landslide factors were assessed including topographic position index, stream power index, slope, aspect, elevation, profile curvature, distance to drainage, soil, rainfall, land use, and distance to road. The assessment of the landslide susceptibility level in this study was highly accurate, based on the AUC value obtained, which was 0.92. The results of the assessment of the landslide susceptibility level were divided into five classes with the following areas: very low 36%, low 4.4%, moderate 2.91%, high 4.1% and very high 52.5%.
Keywords: scientific, approach, methodological, techniques, geography
References:
[1] Asriningrum W, Noviar H, Suwarsono S. Development of zoning method for volcanic eruption hazard areas, case study of Mount Maria. Journal of Remote Sensing and Digital Image Data Processing. 2019; Volume 1(Issue 1):66-75.
[2] Nurhadi N, Ashari A, Suparmini S. Environmental and Infrastructure Characteristics Relation to the Level of Preparedness in the Merapi Eruption Disaster-Prone Areas. Geomedia. 2021; Volume 14 (Issue 2):149-164.
[3] Ahmed B. Landslide susceptibility mapping using multi-criteria evaluation techniques in Chittagong metropolitan area. Bangladesh Landslides. 2015;12(6):1077–95.
[4] Wang LJ, Guo M, Sawada K, Lin J, Zhang J. Landslide susceptibility mapping in Mizunami City, Japan: A comparison between logistic regression, bivariate statistical analysis and multivariate adaptive regression spline models. Catena. 2015;135:271– 82.
[5] Chen W, Pourghasemi HR, Kornejady A, Zhang N. Landslide spatial modeling: Introducing new ensembles of ANN, MaxEnt, and SVM machine learning techniques. Geoderma. 2017;305:314–27.
[6] Pham BT, Tien Bui D, Pourghasemi HR, Indra P, Dholakia MB. Landslide susceptibility assessment in the Uttarakhand area (India) using GIS: A comparison study of prediction capability of nave bayes, multilayer perceptron neural networks, and functional tree methods. Theoretical and Applied Climatology. 2017;128(1–2):255– 73.
[7] James G, Witten D, Hastie T, Tibshirani R. An introduction to statistical learning: With applications. London: Springer; 2017.
[8] Pradhan AM, Kim YT. Evaluation of a combined spatial multi-criteria evaluation model and deterministic model for landslide susceptibility mapping. Catena. 2016;140:125– 39.
[9] Devkota KC, Regmi AD, Yoshida KH et al. Landslide susceptibility mapping using certainty factor, index of entropy and logistic regression models in GIS and their comparison at Mugling-Narayanghat road section in Nepal Himalayas. Natural Hazards. 2013;65(1):135–65.
[10] Umar Z, Pradhan B, Ahmad A, Jebur MN, Tehrany MS. Earthquake induced erosion susceptibility mapping using an integrated ensemble frequency ratio and logistic regression models in West Sumatra Province, Indonesia. Catena. 2014;118:124–35.
[11] Panoto D, Irawan LY, Dahlia S, Herlambang GA, Rasyidah AN. Mapping of flash flood vulnerability in Dau District, Malang Regency using the analytic hierarchy process method. Journal of Georaflesia. 2020;5(2):143–54.
[12] Mandal S, Maiti R. Application of analytical hierarchy process (AHP) and frequency ratio (FR) model in assessing landslide susceptibility and risk. Singapore: Springer; 2015.
[13] Van Zuidam RA. Terrain analysis and classification using aerial photographs: A geomorphological approach. Enschede: International Institute for Aerial Survey and Earth Sciences; 1973.
[14] Bachri S, Sumarmi S, Irawan LY et al. Landslide susceptibility mapping (NGO) in Kelud volcano using spatial multi-criteria evaluation. IOP Conference Series: Earth and Environmental Science. 2019;273(1):1–26.
[15] Irawan LY, Sumarmi S, Bachri S., Panoto D, Pradana IH, Faizal R. Landslide susceptibility mapping based on geospatial data and geomorphic attributes (A case study: Pacet, Mojokerto, East Java). IOP Conference Series: Earth and Environmental Science. 2020 Sep 12. East Java, Indonesia. Available from https://iopscience.iop.org/article/10.1088/1755-1315/747/1/012002/pdf.
[16] Coanji T. Slope analysis based on SRTM digital elevation model data: Case study on Rokan 4 Koto area and surroundings. International Journal of Engineering and High-End Technology. 2016;1:71–75.
[17] Pawluszek K, Borkowski A. Impact of DEM-derived factors and analytical hierarchy process on landslide susceptibility mapping in the region of Rożnów Lake, Poland. Natural Hazards. 2017;86(2):919–52.
[18] Sartohadi J, Jamulya J, Dewi NIS. Introduction to soil geography. Yogyakarta: Student Library; 2012.
[19] Pourghasemi HR, Yansari ZT, Panagos P, Pradhan B. Analysis and evaluation of landslide susceptibility: A review on articles published during 2005–2016 (periods of 2005–2012 and 2013–2016). Arabian Journal of Geosciences. 2018;11(9):193-205.
[20] Jebur MN, Pradhan B, Tehrany MS. Optimization of landslide conditioning factors using very high-resolution airborne laser scanning (LiDAR) data at catchment scale. Remote Sensing of Environment. 2014;152:150–65.
[21] Varnes DJ. Landslide hazard zoning: A review of principles and practice. Paris: The UNESCO Press; 1984.
[22] Das I, Sahoo S, Westen CV, Stein A, Hack R. Landslide susceptibility assessment using logistic regression and its comparison with a rock mass classification system, along a road section in the northern Himalayas (India). Geomorphology. 2010;114(4):627–37.
[23] Chen W, Peng J, Hong H et al. Landslide susceptibility modelling using GIS-based machine learning techniques for Chongren County, Jiangxi Province, China. Science of the Total Environment. 2018;626:1121–35.
[24] Ayalew L, Yamagishi H. The Application of GIS-based logistic regression for landslide susceptibility mapping in the Kakuda-Yahiko Mountains, Central Japan. Geomorphology. 2005;65(1-2):15-31. https://doi.org/10.1016/j.geomorph.2004.06.010
[25] Chen W, Xie X, Peng J, Wang J, Duan Z, Hong H. GIS-based landslide susceptibility modelling: A comparative assessment of kernel logistic regression, Naïve-Bayes tree, and alternating decision tree models. Geomatics, Natural Hazards and Risk. 2017;8(2):950–73.
[26] Pradhan B. Landslide susceptibility mapping of a catchment area using frequency ratio, fuzzy logic and multivariate logistic regression approaches. Journal of the Indian Society of Remote Sensing. 2010;38(2):301–20.
[27] Wang Y, Fang Z, Hong H. Comparison of convolutional neural networks for landslide susceptibility mapping in Yanshan County, China. Science of the Total Environment. 2019;666:975–93.
[28] Hemasinghe H, Rangali RS, Deshapriya NL, Samarakoon L. Landslide susceptibility mapping using logistic regression model (A case study in Badulla District, Sri Lanka). Procedia Engineering. 2018;212:1046–53.
[29] Qiang X, Guang ZHENG, Weile L et al. Study on successive landslide damming events of Jinsha River in Baige Village on Octorber 11 and November 3, 2018. Journal of Engineering Geology. 2018;26(6):1534-1551.
[30] Zhou S, Wang W, Chen G, Liu B, Fang L. A combined weight of evidence and logistic regression method for susceptibility mapping of earthquake induced landslides: A case study of the April 20, 2013 Lushan earthquake, China. China: Acta Geologica Sinica. 2016; 90 (2)-511-524.
[31] Pourghasemi HR, Kariminejad N, Gayen A, Komac M. Statistical functions used for spatial modelling due to assessment of landslide distribution and landscapeinteraction factors in Iran. Geoscience Frontiers. 2020;11(4):1257–69.