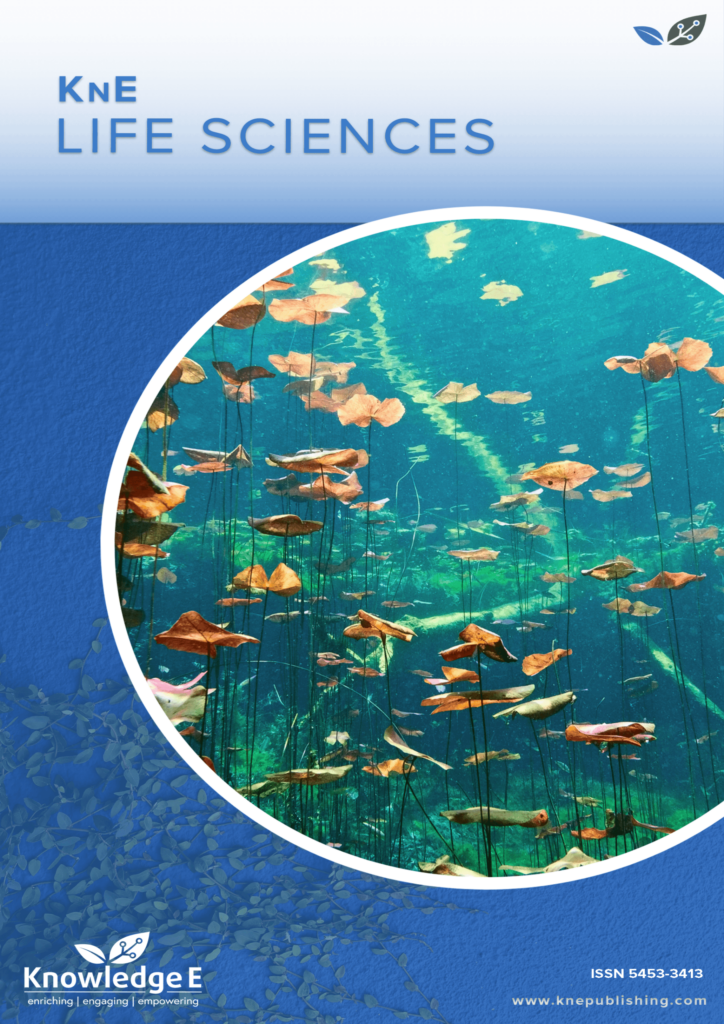
KnE Life Sciences
ISSN: 2413-0877
The latest conference proceedings on life sciences, medicine and pharmacology.
Prediction of Dengue Fever Cases in Malang City using a Neural Network Model
Published date: Mar 25 2021
Journal Title: KnE Life Sciences
Issue title: The 2nd International Scientific Meeting on Public Health and Sports (ISMoPHS 2020)
Pages: 81–92
Authors:
Abstract:
Dengue fever has been declared endemic in many cities of Indonesia, one of them being the Malang City. In 2015, the incidence of dengue fever in the region was recorded at 1,629 with 13 deaths. There are many factors that contribute to the disease. The factors associated with dengue-fever transmission include population density, population mobility, quality of housing and attitude of life. However, the factors that can trigger dengue fever are environmental in nature, and include changes in temperature, humidity and rainfall, which cause mosquitoes to lay eggs more often and facilitates a rapid reproduction of the dengue virus. Parasites and disease carriers (mosquitoes) are very sensitive to climatic factors, especially temperature, rainfall, humidity, water levels and wind. Therefore, this study aimed to develop a suitable model for forecasting dengue fever in Malang City based on the Transfer Function and Artificial Neural Network (ANN). Data used were dengue fever data from 2004 to 2019. The results showed that the smallest RMSE, MAPE and SMAPE values of the two models were ANN models.
Keywords: Artificial Neural Network (ANN), transfer function, dengue fever
References:
[1] Gao, Y., et al. (2020). Deep Learning and Transfer Learning Models of Energy Consumption Forecasting for a Building with Poor Information Data. Energy & Buildings, vol. 223, issue 1, pp. 110-6.
[2] Güven, İ. and Simsir, F. (2020). Demand Forecasting with Color Parameter in Retail Apparel Industry Using Artificial Neural Networks (ANN) and Support Vector Machines (SVM) Methods. Computers & Industrial Engineering, vol. 147, issue 1, pp. 1-10.
[3] Hardiana, V. M. D. (2013). Susilaningrum, and Suhartono, Peramalan Jumlah Tamu di Hotel “X” dengan Pendekatan Arima, Fungsi Transfer, dan Anfis. Jurnal Sains Dan Seni Pomits, vol. 2, issue 2, pp. 183-8.
[4] Jia, B., Dong, R. and Du, J. (2020). Ozone Concentrations Prediction in Lanzhou, China, using Chaotic Artificial Neural Network. Chemometrics and Intelligent Laboratory Systems, vol. 204, issue 1, pp. 1-8.
[5] Jiang, H., et al. (2020). Prediction of Output Power with Artificial Neural Network using Extended Datasets for Stirling Engines. Applied Energy, issue 271, pp. 115-23.
[6] Kashani, H. R. K., et al. (2020). Prediction Value of Preoperative Findings on Meningioma Grading Using Artificial Neural Network. Clinical Neurology and Neurosurgery, vol. 196, issue 20, pp. 1-7.
[7] Ma’rufah, N., Rahayu, S.P. and Suhartono. (2013). Peramalan Pendapatan Operasional Bank Menggunakan Metode Fungsi Transfer dan Neural Network. Jurnal Sains Dan Seni Pomits, vol. 2, issue 2, pp. 219-24.
[8] Nurina, D. L. and Irhamah. (2013). Peramalan Volume Pemakaian Air Sektor Rumah Tangga di Kabupaten Gresik dengan Menggunakan Fungsi Transfer. Jurnal Sains Dan Seni Pomits, vol. 2, issue 2, pp. 260-4.
[9] Nurvitasari, Y. and Irhamah. (2012). Pendekatan Fungsi Transfer Sebagai Input Adaptive Neuro-Fuzzy Inference System (ANFIS) dalam Peramalan Kecepatan Angin Rata-Rata Harian di Sumenep. Jurnal Sains Dan Seni ITS, vol. 1, issue 1, pp. 62-8.
[10] Ye, R. and Dai, Q. (2020). Implementing Transfer Learning across Different Datasets for Time Series Forecasting Pattern Recognition 2021. Pattern Recognition, vol. 109, issue 1, pp. 1-6.