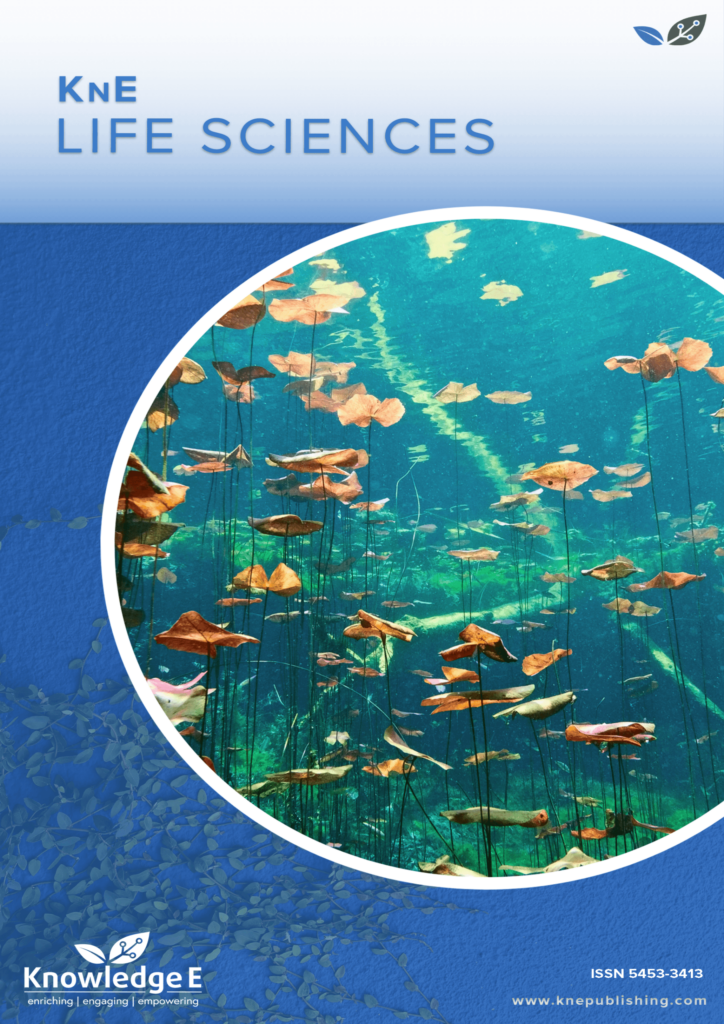
KnE Life Sciences
ISSN: 2413-0877
The latest conference proceedings on life sciences, medicine and pharmacology.
COVID-19 Death Risk in Surabaya: Modeling by Spatial Point Process
Published date: Mar 27 2024
Journal Title: KnE Life Sciences
Issue title: International Conference On Mathematics And Science Education (ICMScE 2022): Life Sciences
Pages: 206–217
Authors:
Abstract:
The total death rate or Case Fatality Rate (CFR) due to COVID-19 in Surabaya is high, that is almost twice of the global CFR (1.4%). Utilization of high-resolution data has the potential to explore COVID-19 cases, not only recording cases at the district or city level but also at the patient’s domicile level so that they can provide more detailed spatial information. Meanwhile, research exploring the risk of death from COVID-19, especially in Surabaya using spatial point process model, has not yet been carried out. In this study, an analysis of the risk of death from COVID-19 in Surabaya will be carried out using the inhomogeneous Poisson point process model with covariates or external factors used including the density of the COVID-19 referral hospital location and the proportion of confirmed COVID-19 population aged > 60 years per districts. Our model shows that referral hospitals (exp( ) = 1.03295) and places of worship (exp( ) = 1.03835) have a significant effect on death risk from COVID-19. So, there is a need for special handling for areas that have a population with a vulnerable age (> 60 years) where at this age the human immune system will decrease.
Keywords: COVID-19, heath risk, spatial point process, surabaya
References:
[1] W.H.O. “Novel corona virus,” https://www.who.int/indonesia/news/novel-coronavirus.
[2] S.C. -19 Jatim, “Jatim tanggap Covid-19,” https://infocovid19.jatimprov.go.id/
[3] Park J, Chang W, Choi B. An interaction Neyman-Scott point process model for coronavirus disease-19. Spat Stat. 2022 Mar;47(47):100561.
[4] A. Choiruddin, F. Hannanu, J. Mateu, and V. Fitriyanah, “COVID-19 transmission risk in Surabaya and Sidoarjo: An inhomogeneous marked Poisson point process approach,” (2022). https://doi.org/10.21203/rs.3.rs-2258695/v1.
[5] Virania TA, Choiruddin A, Ratnasari V. Analisis risiko penyebaran kasus Covid-19 di Surabaya Raya menggunakan model thomas cluster process. Inferensi. 2021;4(1):57.
[6] Izati PP, Choiruddin A. Pemodelan risiko penyebaran COVID-19 di Surabaya Raya menggunakan model cauchy cluster process. Inferensi. 2022;5(1):1.
[7] Nurhayatun S, Fitriyanti N, Sonia D. Analisis Average Length of Stay (AVLOS) kasus covid-19 di rumah sakit x Bandung. Jurnal Ilmiah Manusia Dan Kesehatan. 2021;4(3):412–8.
[8] S.&. N. Tamher, Kesehatan usia lanjut dengan pendekatan asuhan keperawatan. Salemba Medika, Jakarta, 2009.
[9] Luo Y, Yan J, McClure S. Distribution of the environmental and socioeconomic risk factors on COVID-19 death rate across continental USA: a spatial nonlinear analysis. Environ Sci Pollut Res Int. 2021 Feb;28(6):6587–99.
[10] Elson R, Davies TM, Lake IR, Vivancos R, Blomquist PB, Charlett A, et al. The spatiotemporal distribution of COVID-19 infection in England between January and June 2020. Epidemiol Infect. 2021 Mar;149:e73.
[11] P. Purwanto, S. Utaya, B. Handoyo, et al., “Spatiotemporal analysis of COVID-19 spread with emerging hotspot analysis and space-time cube models in East Java, Indonesia.,” ISPRS International Journal of Geo-Information. vol. 10, no. 3, p. 2021. https://doi.org/10.3390/ijgi10030133.
[12] Baddeley A, Rubak E, Turner R. Spatial point patterns methodology and applications with R. Boca Raton: CRC Press; 2015. https://doi.org/10.1201/b19708.
[13] J. Møller and R.P. Waagepetersen, “Modern statistics for spatial point processes.,” Scandinavian Journal of Statistics. vol. 4, no. 34, pp. 070927154002001-???, 2007. https://doi.org/10.1111/j.1467-9469.2007.00569.x.
[14] Choiruddin A, Coeurjolly J, Waagepetersen R. Information criteria for inhomogeneous spatial point processes. Aust N Z J Stat. 2021;63(1):119–43.
[15] Ripley B. Modelling spatial patterns. J R Stat Soc B. 1977;39(2):172–92.
[16] Berman M, Turner TR. Approximating point process likelihoods with GLIM. Appl Stat. 1992;41(1):31.