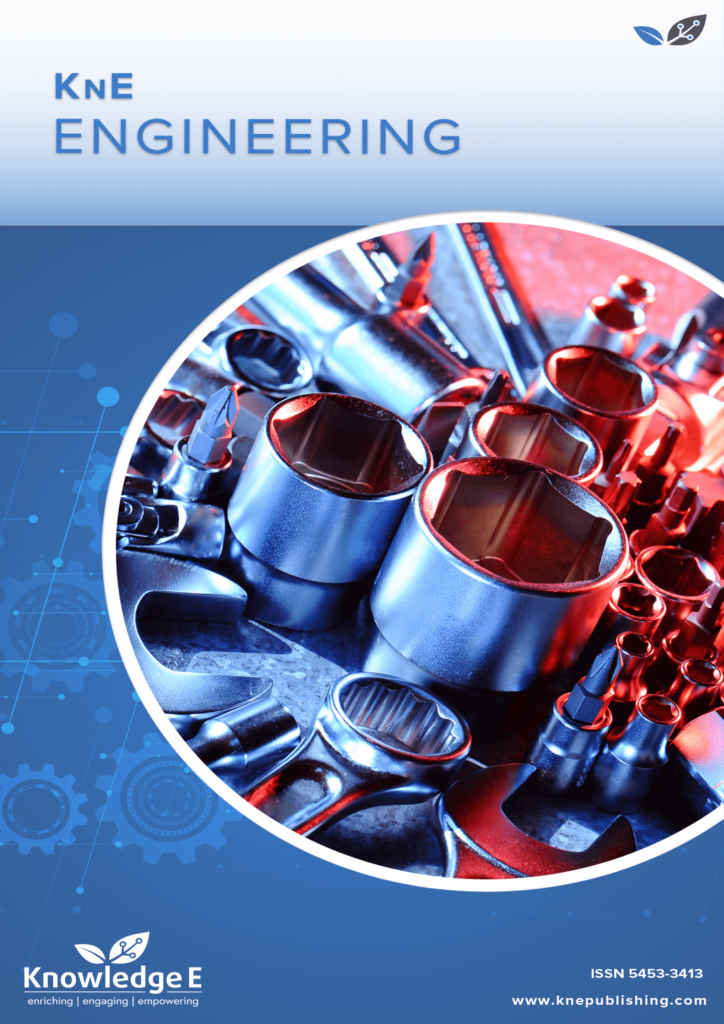
KnE Engineering
ISSN: 2518-6841
The latest conference proceedings on all fields of engineering.
Implementation of Polynomial Functions to Improve the Accuracy of Machine Learning Models in Predicting the Corrosion Inhibition Efficiency of Pyridine-Quinoline Compounds as Corrosion Inhibitors
Published date: Mar 07 2024
Journal Title: KnE Engineering
Issue title: Jakarta International Conference on Multidisciplinary Studies towards Creative Industries (JICOMS)
Pages: 78–87
Authors:
Abstract:
Historically, the exploration of corrosion inhibitor technology has relied extensively on experimental methodologies, which are inherently associated with substantial costs, prolonged durations, and significant resource utilization. However, the emergence of ML approaches has recently garnered attention as a promising avenue for investigating potential materials with corrosion inhibition properties. This study endeavors to enhance the predictive capacity of ML models by leveraging polynomial functions. Specifically, the investigation focuses on assessing the effectiveness of pyridinequinoline compounds in mitigating corrosion. Diverse ML models were systematically evaluated, integrating polynomial functions to augment their predictive capabilities. The integration of polynomial functions notably amplifies the predictive accuracy across all tested models. Notably, the SVR model emerges as the most adept, exhibiting R² of 0.936 and RMSE of 0.093. The outcomes of this inquiry underscore a significant enhancement in predictive accuracy facilitated by the incorporation of polynomial functions within ML models. The proposed SVR model stands out as a robust tool for prognosticating the corrosion inhibition potential of pyridine-quinoline compounds. This pioneering approach contributes invaluable insights into advancing machine learning methodologies geared toward designing and engineering materials with promising corrosion inhibition properties.
Keywords: machine learning, polynomial, corrosion inhibition, pyridine-quinoline
References:
[1] Abdoli L, Huang J, Li H. Electrochemical corrosion behaviors of aluminum-based marine coatings in the presence of Escherichia coli bacterial biofilm. Mater Chem Phys 2016;173.
[2] Akrom M, Saputro AG, Maulana AL, Ramelan A, Nuruddin A, Rustad S, Dipojono HK. DFT and microkinetic investigation of oxygen reduction reaction on corrosion inhibition mechanism of iron surface by Syzygium Aromaticum extract. Appl Surf Sci 2023;615:156319.
[3] Anadebe VC et al. Multidimensional insight into the corrosion inhibition of salbutamol drug molecule on mild steel in oilfield acidizing fluid: Experimental and computer aided modeling approach. J Mol Liq 2022;349.
[4] Ivoševic Š, Kovac N, Vastag G, Majeric P, Rudolf R. A probabilistic method for estimating the influence of corrosion on the cualni shape memory alloy in different marine environments. Crystals 2021;11:3.
[5] Mythreyi OV, Srinivaas MR, Amit Kumar T, Jayaganthan R. Machine-learning-based prediction of corrosion behavior in additively manufactured inconel 718. Data 2021;6:8.
[6] Haladu SA, Dalhat Mu’azu N, Ali SA, Elsharif AM, Odewunmi NA, Abd El-Lateef HM. Inhibition of mild steel corrosion in 1 M H2SO4 by a gemini surfactant 1,6-hexyldiylbis-( dimethyldodecylammonium bromide): ANN, RSM predictive modeling, quantum chemical and MD simulation studies. J Mol Liq 2022;350.
[7] Verma C, Quraishi MA, Ebenso EE. Quinoline and its derivatives as corrosion inhibitors: A review. Surfaces and Interfaces 2020;21:100634.
[8] Zhang W, Li H, Wang Y, Liu Y, Wu Y. Adsorption and corrosion inhibition properties of pyridine-2-aldehyde-2-quinolylhydrazone for Q235 steel in acid medium: Electrochemical, thermodynamic, and surface studies. Materials and Corrosion 2018;69:11:1638-1648.
[9] Hau NN, Huong DQ. Effect of aromatic rings on mild steel corrosion inhibition ability of nitrogen heteroatom-containing compounds: Experimental and theoretical investigation. Journal of Molecular Structure 2013;1277:134884.
[10] Ayyannan G, Karthikeyan K, Vivekananthan SS, Gopiraman M, Rathinavelu A. Chemical and electrochemical investigations of high carbon steel corrosion inhibition in 10 % HCl medium by quinoline chalcones. Ionics 2013;19:919.
[11] Daoud D, Hamani H, Douadi T. Novel heterocyclic quinoline derivatives as green environmental corrosion inhibitors for carbon steel in HCl solution: experimental and theoretical investigation. Journal of Adhesion Science and Technology 2021;35:21.
[12] Lgaz H, Salghi R, Bhat KS, Chaouiki A, Shubhalaxmi, Jodeh S. Correlated experimental and theoretical study on inhibition behavior of novel quinoline derivatives for the corrosion of mild steel in hydrochloric acid solution. Journal of Molecular Liquids 2017;244:154.
[13] Obot IB, Macdonald DD, Gasem ZM. Density functional theory (DFT) as a powerful tool for designing new organic corrosion inhibitors: Part 1: An overview. Corrosion Science 2015;99.
[14] Khaled KF, Al-Mobarak NA. A predictive model for corrosion inhibition of mild steel by thiophene and its derivatives using artificial neural network. Int J Electrochem Sci 2012;7:2:1045-1059.
[15] Zhao H, Zhang X, Ji L, Hu H, Li Q. Quantitative structure-activity relationship model for amino acids as corrosion inhibitors based on the support vector machine and molecular design. Corros Sci 2014;83:261–271.
[16] Liu Y et al. A Machine Learning-Based QSAR Model for Benzimidazole Derivatives as Corrosion Inhibitors by Incorporating Comprehensive Feature Selection. Interdiscip Sci Comput Life Sci 2019;11:4:738–747.
[17] Akrom M, Rustad S, Saputro AG, Fathurrahman F, Dipojono HK. A Combination of Machine Learning Model and Density Functional Theory Method to Predict Corrosion Inhibition Performance of New Diazine Derivative Compounds. Mat Today Comm 2023;35.
[18] Quadri TW et al. Development of QSAR-based (MLR/ANN) predictive models for effective design of pyridazine corrosion inhibitors. Mater Today Commun 2022;30:103163.
[19] Ser CT, Žuvela P, Wong MW. Prediction of corrosion inhibition efficiency of pyridines and quinolines on an iron surface using machine learning-powered quantitative structure-property relationships. Appl Surf Sci 2020;512.
[20] Yasmin NSA, Wahab NA, Anuar AN. Improved support vector machine using optimization techniques for an aerobic granular sludge. Bull Electr Eng Informatics 2020;9:5.
[21] Akrom M, Rustad S, Saputro AG, Dipojono HK. Data-driven investigation to model the corrosion inhibition efficiency of Pyrimidine-Pyrazole hybrid corrosion inhibitors. Comp and Theo Chem 2023;1229:114307.
[22] Beltran-Perez C et al. A General Use QSAR-ARX Model to Predict the Corrosion Inhibition Efficiency of Drugs in Terms of Quantum Mechanical Descriptors and Experimental Comparison for Lidocaine. Int J Mol Sci 2022;23:9.
[23] Skrypnik YG, Doroshenko TF, Skrypnik SY. On the influence of the nature of substituents on the inhibiting activity of meta- and para-substituted pyridines. Mater Sci 1996;31:3:324–330.
[24] Doroshenko TV, Lyashchuk SN, Skrypnik YG. The HSAB principle in the description of the inhibitive effectiveness of heterocyclic N-bases. Prot Met 2000;36:3:244–247.
[25] Akrom M, Rustad S, Dipojono HK. Machine learning investigation to predict corrosion inhibition capacity of new amino acid compounds as corrosion inhibitors. Result in Chem 2023;6:101126.
[26] Shahini MH, Keramatinia M, Ramezanzadeh M, Ramezanzadeh B, Bahlakeh G. Combined atomic-scale/DFT-theoretical simulations & electrochemical assessments of the chamomile flower extract as a green corrosion inhibitor for mild steel in HCl solution. J Mol Liq 2021;342:117570.
[27] Chen X, Chen Y, Cui J, Li Y, Liang Y, Cao G. Molecular dynamics simulation and DFT calculation of ‘green’ scale and corrosion inhibitor. Comput Mater Sci 2020;188:110229.
[28] Kokalj A. Corrosion inhibitors: physisorbed or chemisorbed?. Corros Sci 2022;196:109939.
[29] Kozlica DK, Kokalj A, Milošev I. Synergistic effect of 2-mercaptobenzimidazole and octylphosphonic acid as corrosion inhibitors for copper and aluminium – An electrochemical, XPS, FTIR and DFT study. Corros Sci 2021;182.
[30] Buitinck L et al. API design for machine learning software: experiences from the scikit-learn project. 2013;1–15.
[31] Alamri AH, Alhazmi N. Development of data driven machine learning models for the prediction and design of pyrimidine corrosion inhibitors. Journal of Saudi Chemical Society 2022;26:6.
[32] Quadri TW et al. Computational insights into quinoxaline-based corrosion inhibitors of steel in HCl: Quantum chemical analysis and QSPR-ANN studies. Arabian Journal of Chemistry 2022;15:7.
[33] El Assiri EH et al. Development and validation of QSPR models for corrosion inhibition of carbon steel by some pyridazine derivatives in acidic medium. Heliyon 2020;6:10.
[34] Obot IB, Umoren SA. Experimental, DFT and QSAR models for the discovery of new pyrazines corrosion inhibitors for steel in oilfield acidizing environment. Int J Electrochem Sci 2020;15:9:9066–9080.