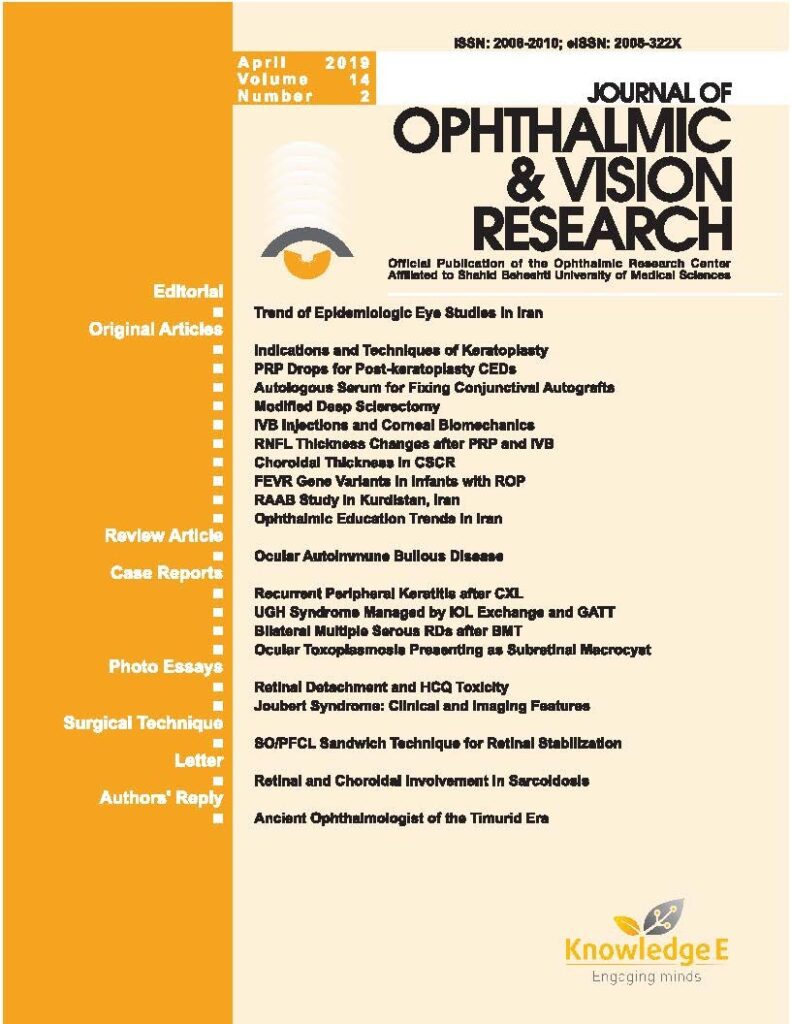
Journal of Ophthalmic and Vision Research
ISSN: 2008-322X
The latest research in clinical ophthalmology and the science of vision.
Editorial – Application of Artificial Intelligence to Improve Imaging in Ophthalmology
Published date: Feb 13 2023
Journal Title: Journal of Ophthalmic and Vision Research
Issue title: Jan–Mar 2023, Volume 18, Issue 1
Pages: 1 – 2
Authors:
Abstract:
This is an Editorial and does not have an abstract. Please download the PDF or view the article in HTML.
Keywords: Editorial
References:
1. Emmert-Streib F, Yang Z, Feng H, Tripathi S, Dehmer M. An introductory review of deep learning for prediction models with big data. Front Artif Intell 2020;3:4.
2. Aggarwal R, Sounderajah V, Martin G, Ting DSW, Karthikesalingam A, King D, et al. Diagnostic accuracy of deep learning in medical imaging: A systematic review and meta-analysis. NPJ Digit Med 2021;4:65.
3. Ting DSW, Pasquale LR, Peng L, Campbell JP, Lee AY, Raman R,et al. Artificial intelligence and deep learning in ophthalmology. Br J Ophthalmol 2019;103:167–175.
4. FDA. FDA permits marketing of artificial intelligence based device to detect ceretain diabetes-related eye problems. Accessed January 10, 2022. https: //www.fda.gov/news-events/press-announcements/fdapermits- marketing-artificial-intelligence-based-devicedetect-certain-diabetes-related-eye
5. Lee KJ. Autonomous diabetic retinopathy screening system gains FDA approval. Accessed December 20, 2022. https://www.aao.org/headline/autonomousdiabetic-retinopathy-screening-system-g
6. Razaghi G, Aghsaei M, Hejazi M. Correction of retinal nerve fiber layer thickness determination on spectral domain optical coherence tomographic images using Unet architecture. J Ophthalmic Vis Res 2023;18:1–11.
7. Mansberger SL, Menda SA, Fortune BA, Gardiner SK, Demirel S. Automated segmentation errors when using optical coherence tomography to measure retinal nerve fiber layer thickness in glaucoma. Am J Ophthalmol 2017;174:1–8.
8. Miki A, Kumoi M, Usui S, Endo T, Kawashima R, Morimoto T, et al. Prevalence and associated factors of segmentation errors in the peripapillary retinal nerve fiber layer
and macular ganglion cell complex in spectral-domain optical coherence tomography images. J Glaucoma 2017;26:995–1000.
9. Devalla SK, Chin KS, Mari JM, Tun TA, Strouthidis NG, Aung T, et al. A deep learning approach to digitally stain optical coherence tomography images of the optic nerve head. Invest Ophthalmol Vis Sci 2018;59:63–74.
10. Wilson M, Chopra R, Wilson MZ, Cooper C, MacWilliams P, Liu Y, et al. Validation and clinical applicability of whole volume automated segmentation of optical coherence tomography in retinal disease using deep learning. JAMA Ophthalmol 2021;139:964–973.
11. Marques R, Andrade De Jesus D, Barbosa-Breda J, Eijgen JV, Stalmans I, Walsum T, et al. Automatic segmentation of the optic nerve head region in optical coherence tomography: A methodological review. Comput Methods Programs Biomed 2022;220:106801.
12. Ronneberger O, Fischer P, Brox T. U-net: Convolutional networks for biomedical image segmentation. 2015:arXiv:1505.04597. https://ui.adsabs.harvard.edu/abs/2015arXiv150504597R