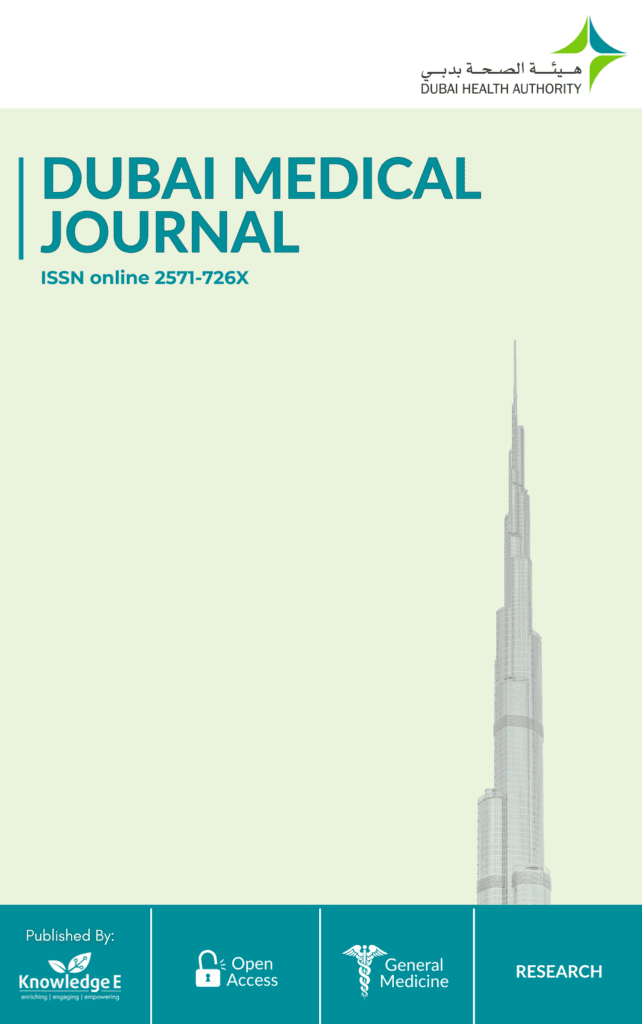
Dubai Medical Journal
ISSN: 2571-726X
Pioneering research in medicine, health sciences, nursing, pharmaceuticals, and laboratory work
A New Approach for Brain Tumor Detection Using Machine Learning
Published date: Dec 08 2024
Journal Title: Dubai Medical Journal
Issue title: Dubai Medical Journal (DMJ): Volume 7 Issue 3
Pages: 160 - 176
Authors:
Abstract:
Introduction: The abnormal brain cells consist of brain tumor which leads to severe organ dysfunction and potentially death. These tumors exhibit a wide range of sizes, textures, and locations. Diagnosing brain tumors process is a time-consuming process requiring the expertise of radiologists. Brain tumors are classified as glioma, meningioma, pituitary, and no tumor. As patient numbers and data volumes rise, traditional methods have become costly and inefficient.
Methods: Researchers have developed algorithms for detecting and classifying brain tumors and prioritizing accuracy and efficiency. Deep learning (DL) techniques are increasingly used to create automated systems capable of precisely diagnosing or segmenting brain tumors, particularly for brain cancer classification. This approach supports the use of transfer learning models in medical imaging. This proposed model is a modification to components of Xception model by adding a lot of parameters for increasing the Xception model efficiency.
Results: This proposed Xception model was applied to Masoud Nickparvar braintumor- mri-dataset, achieving an accuracy of 99.6%, sensitivity of 99.7%, and specificity of 99.7% with an F1 score of 99.9%.
Discussion: The efficiency parameters of the proposed model assured that it is an effective model for diagnosing brain tumor. Comparative analysis with other models shows that the proposed framework is highly reliable for the timely detection of various brain tumors.
Conclusion: The results confirm the effectiveness of our proposed model, which attains higher overall accuracy in tumor detection compared to previous models. As a result, the proposed model is considered a valuable decision-making tool for experts in diagnosing brain tumor.
Keywords: machine learning, deep learning, Xception model, brain tumor, MRI
References:
[1] Zahoor MM, Qureshi SA, Bibi S, Khan SH, Khan A, Ghafoor U, et al. A new deep hybrid boosted and ensemble learning-based brain tumor analysis using MRI. Sensors (Basel). 2022 Apr;22(7):2726.
[2] Lee DY. Roles of mTOR signaling in brain development. Exp Neurobiol. 2015 Sep;24(3):177–185.
[3] Gore DV, Deshpande V. “Comparative study of various techniques using deep Learning for brain tumor detection”, In: 2020, Proc. of the IEEE International Conference for Emerging Technology (INCET), Belgaum, India, pp. 1–4, 5–7 June 2020.
[4] Hollon TC, Pandian B, Adapa AR, Urias E, Save AV, Khalsa SS, et al. Near real-time intraoperative brain tumor diagnosis using stimulated Raman histology and deep neural networks. Nat Med. 2020 Jan;26(1):52–58.
[5] Saeed F, Paul A, Karthigaikumar P, Nayyar AJ. Convolutional neural network based early fire detection. Multimedia Tools Appl. 2020;79(13):9083–9099.
[6] Kwon O, Yoo S. Interoperability reference models for applications of artificial intelligence in medical imaging. Appl Sci. 2021;11(6):2704. https://doi.org/10.3390/app11062704
[7] Wang B, Zhang Y, Wu C, Wang F. Multimodal mri analysis of cervical cancer on the basis of artificial intelligence algorithm. Contrast Media Mol Imaging. 2021 Nov;2021:1673490.
[8] Alwiyah A. A comprehensive survey of machine learning applications in medical image analysis for artificial vision. Int Trans Art Intel (Italic):2(1):90–98. https://doi.org/10.33050/italic.v2i1.438.
[9] Song K. Current status of deep learning applications in abdominal ultrasonography. Ultrasonography. 40(2):177–182. https://doi.org/10.14366/usg.20085
[10] Zhou L, Wang J, Yu S, Wu G, Wei Q, Deng Y, et al. Artificial intelligence in medical imaging of the liver. World J Gastroenterol. 2019;25(6):672–682. https://doi.org/10.3748/wjg.v25.i6.672
[11] Almeida M. The use of artificial intelligence in the classification of medical images of brain tumors. Biomed J Sci Tech Res. 2023;53(4). https://doi.org/10.26717/BJSTR.2023.53.008450
[12] Sukegawa S, Yoshii K, Hara T, Yamashita K, Nakano K, Yamamoto N, et al. Deep neural networks for dental implant system classification. Biomolecules. 2020 Jul;10(7):984.
[13] Barragán-Montero A, Javaid U, Valdés G, Nguyen D, Desbordes P, Macq B, et al. “Artificial intelligence and machine learning for medical imaging: A technology review.” Elsevier BV, Physica Medica. 2021 Mar;83:242–256. https://doi.org/10.1016/j.ejmp.2021.04.016
[14] Abdelhafiz D, Yang C, Ammar R, Nabavi S. “Deep convolutional neural networks for mammography: Advances, challenges and applications.” BMC Bioinformatics, Springer Science and Business Media LLC. 2019 Jun;20:S11. https://doi.org/10.1186/s12859-019-2823-4
[15] Boubacar Goga A. “Artificial intelligence at the service of medical imaging in the detection of breast tumors.” Artificial Intelligence. IntechOpen, Jan 25, 2023. https://doi.org/10.5772/intechopen.108739
[16] Tran WT, Jerzak K, Lu FI, Klein J, Tabbarah S, Lagree A, et al. “Personalized breast cancer treatments using artificial intelligence in radiomics and pathomics.” Elsevier BV, J Med Imag Radiat Sci. 2019 Dec;50(4):S32–S41 https://doi.org/10.1016/j.jmir.2019.07.010
[17] Syeda H, Syed M, Sexton K, Syed S, Begum S, Syed F, et al. Role of machine learning techniques to tackle the covid-19 crisis: Systematic review. Jmir Med Informatics. 2021;9(1):e23811. https://doi.org/10.2196/23811
[18] Xu H, Chen J, Tang T, Shan Y. Cultivation path of compound talents in ophthalmic diagnosis, treatment, and nursing based on artificial intelligence. J Clin Nurs Res. 2022;6(5):106–111. https://doi.org/10.26689/jcnr.v6i5.4387
[19] Simonyan K, Zisserman AJ. “Very deep convolutional networks for large-scale image recognition”. p. arXiv:1409.1556. Accessed on: September 01, 2014.
[20] He K, Zhang X, Ren S, Sun J. “Deep residual learning for image recognition”. 2016 IEEE Conference on Computer Vision and Pattern Recognition (CVPR), Las Vegas, NV, USA, pp. 770–778, 2016.
[21] Szegedy C, Liu W, Jia Y, Sermanet P, Reed S, Anguelov D, et al. “Going deeper with convolutions”. In: Proc. The IEEE Conference on Computer Vision and Pattern Recognition, pp. 1–9, 2015.
[22] Muhammad K, Khan S, Del Ser J, Albuquerque VHC. “Deep learning for multigrade brain tumor classification in smart healthcare systems: A prospective survey”. IEEE Transactions on Neural Networks and Learning Systems, pp. 1-16, 06/30 2020.
[23] Nodirov J, Abdusalomov AB, Whangbo TK. Attention 3D U-Net with multiple skip connections for segmentation of brain tumor images. Sensors (Basel). 2022 Aug;22(17):6501.
[24] Abd-Ellah MK, Awad AI, Khalaf AA, Hamed HF. A review on brain tumor diagnosis from MRI images: Practical implications, key achievements, and lessons learned. Magn Reson Imaging. 2019 Sep;61:300– 318.
[25] Yushkevich PA, Yang Gao, Gerig G. ITK-SNAP: An interactive tool for semi-automatic segmentation of multi-modality biomedical images. Annu Int Conf IEEE Eng Med Biol Soc. 2016 Aug;2016:3342–3345.
[26] Latif G, Brahim G, Iskandar D, Bashar A, Alghazo J. “Glioma tumors’ classification using deep-neural-network-based features with SVM classifier”. Diagnostics. 2022;12:1018. https://doi.org/10.3390/diagnostics12041018
[27] Hossain T, Shishir FS, Ashraf M, Nasim MA, Shah FM. “Brain tumor detection using convolutional neural network”. In: Proc. 1st International Conference on Advances in Science, Engineering and Robotics Technology (ICASERT), pp. 1–6, 2019.
[28] Khan AR, Khan S, Harouni M, Abbasi R, Iqbal S, Mehmood Z. Brain tumor segmentation using K-means clustering and deep learning with synthetic data augmentation for classification. Microsc Res Tech. 2021 Jul;84(7):1389–1399.
[29] Yahyaoui H, Ghazouani F, Farah IR. “Deep learning guided by an ontology for medical images classification using a multimodal fusion”, In: proc. International Congress of Advanced Technology and Engineering (ICOTEN), pp. 1–6, 2021.
[30] Kang J, Ullah Z, Gwak J. MRI-based brain tumor classification using ensemble of deep features and machine learning classifiers. Sensors (Basel). 2021 Mar;21(6):2222.
[31] Bhatele KR, Bhadauria SS. Machine learning application in Glioma classification: Review and comparison analysis. Arch Comput Methods Eng. 2022;29(1):247–74.
[32] Swati ZN, Zhao Q, Kabir M, Ali F, Ali Z, Ahmed S, et al. Brain tumor classification for MR images using transfer learning and fine-tuning. Comput Med Imaging Graph. 2019 Jul;75:34–46.
[33] Mehmood M, Alshammari N, Alanazi SA, Basharat A, Ahmad F, Sajjad M, et al. Improved colorization and classification of intracranial tumor expanse in MRI images via hybrid scheme of Pix2Pix-cGANs and NASNet-large. J King Saud Univ Comput Inf Sci. 2022;34(7):4358–4374.
[34] Sartaj. Brain tumor classification dataset. Available online: https://www.kaggle.com/datasets/ sartajbhuvaji/brain-tumorclassification-mri.
[35] Nickparvar M. Brain tumor classification dataset. Available online: https://www.kaggle.com/datasets/ masoudnickparvar/brain-tumor-mri-dataset.
[36] Chollet F. Xception: Deep learning with depthwise separable convolutions. https://doi.org/10.1109/ CVPR.2017.195
[37] Martins J, Junior J, Pätzig M, Sant’Ana D, Pistori H, Liesenberg V, et al. Identifying plant species in kettle holes using uav images and deep learning techniques. Remote Sens Ecol Conserv. 2022;9(1):1– 16. https://doi.org/https://doi.org/10.1002/rse2.291
[38] Sreevidya Nelli, et al. Revolutionizing breast cancer detection: Enhanced mammogram analysis with modified Xception and self-attention. Int J Recent Innov Trends Comp Comm. 2023;11(11):484–490. https://doi.org/10.17762/ijritcc.v11i11.9950
[39] Abunasser BS, AL-Hiealy MRJ, Zaqout IS, Abu-Naser SS. “Breast cancer detection and classification using deep learning Xception algorithm.” Int J Adv Comp Sci Appl 2022;13(7). The Science and Information Organization. https://doi.org/10.14569/IJACSA.2022.0130729
[40] Khan AI, Shah JL, Bhat MM. “CoroNet: A deep neural network for detection and diagnosis of COVID-19 from chest x-ray images.” Elsevier BV, Comp Methods Prog Biomed. 2020 Nov;196:105581. https://doi.org/10.1016/j.cmpb.2020.105581
[41] Ayadi M, Ksibi A, Al-Rasheed A, Soufiene BO. “COVID-AleXception: A deep learning model based on a deep feature concatenation approach for the detection of COVID-19 from Chest X-ray Images.” MDPI AG, Healthcare. 2022 Oct;10(10):2072. https://doi.org/10.3390/healthcare10102072
[42] Kwon KW, Park SH, Lee DH, Kim DY, et al. “Deep learning algorithm for the automated detection and classification of nasal cavity mass in nasal endoscopic images.” PLOS ONE. 2024 Mar;19(3):e0297536. https://doi.org/10.1371/journal.pone.0297536
[43] Murthy MY, Koteswararao A, Babu MS. Adaptive fuzzy deformable fusion and optimized CNN with ensemble classification for automated brain tumor diagnosis. Biomed Eng Lett. 2021 Nov;12(1):37–58.
[44] Shah HA, Saeed F, Yun S, Park JH, Paul A, Kang JM. A robust approach for brain tumor detection in magnetic resonance images using finetuned EfficientNet. IEEE Access. 2022;10:65426–65438.
[45] Montalbo FJ. A computer-aided diagnosis of brain tumors using a fine-tuned YOLO-based model with transfer learning. KSII Trans Internet Inf Syst. 2021 Jan;14:4816–4834.